下载中心
优秀审稿专家
优秀论文
相关链接
摘要
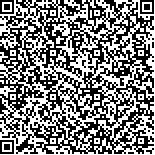
现有密集匹配点云数据已实现了地表3维信息的精细化表达,然而由于误匹配,此类点云往往包含一定数量粗差点并影响后续应用的处理效果。针对此类数据中误匹配所产生粗差点的剔除问题,将变差函数引入移动最小二乘(MLS)粗差剔除算法。变差函数对MLS拟合区域内点对间的相关性进行估算,以此为依据设置权值对最小二乘的结果进行优化;然后利用MLS局部、分区域地对点云进行曲面拟合;最终根据拟合结果剔除粗差。利用A3数字测图系统生成的城区、山区密集点云数据进行实验,并将处理结果、等权MLS处理结果与人工剔除结果进行对比。实验结果表明该算法可有效对点云中的粗差进行剔除,相较于等权MLS粗差剔除算法,该算法在城区、山区的误判率分别降低了5.16%和1.31%。
With the development of digital mapping cameras and image-matching techniques, highly dense point clouds can be automatically obtained by stereo image matching. These point clouds can be applied to 3D building reconstruction and DEM/DSM generation. However, owing to certain factors, such as textural differences, geometric distortions, and shadows, point clouds sustain gross errors. To address this problem, a semivariogram-based weight moving least square (SWMLS) method is proposed for gross error detection in point clouds obtained by stereo image matching.
The SWMLS method is an iterative process. For each iteration, a semivariogram is used to estimate in advance the covariance between points in different distances and aspects. The weight can be calculated based on the estimated covariance and applied to approximate the least square and terrain surface separately. The distance between each point and the surface is calculated with the approximated local parametric surface, and the distance-constrained histogram method is used to detect the gross errors. Ingross error detection, the least square approximation move through the entire region of the point cloud by the same steps in the x-axis and y-axis. In this paper, we focus on discussing the adaptability of semivariogram to the digital elevation model and its variation across different terrains.
The A3 system-generated point clouds of two datasets of urban and mountainous area ares used in the experiments. The point cloud for the urban area contains a 4.00% gross error, whereas the point cloud for the mountainous area contains 0.19% gross error. To test the efficiency and advantages of our method, the results of our method and those of the equal weight based MLS (EWMLS) method are compared manually to the detected results. Experimental results show that both SWMLS and EWMLS have removed all the gross errors in the point cloud. However, the results of a further quantitative analysis suggest that SWMLS is more accurate than EWMLS. For the urban area, the misclassification by S WMLS is 2.07%, whereas that by EWMLS is 7.72%. For the mountainous area, EWMLS has committed 2.56% misclassification, whereas our proposed method generated a reduced misclassification (0.76%).
We may draw the conclusion as below. (1) As a regionalized method, semivariogram is efficient in weight estimation in MLS. (2)Both SWMLS and EWMLS are effective in detecting gross errors in point clouds generated from stereo images. However, both methods may commit few misclassifications for urban and mountainous areas. (3)Compared with EWMLS, SWMLS generated less misclassification in both datasets, suggesting that SWMLS demonstrates a better performance in the gross error detection in stereo-matched point clouds.