下载中心
优秀审稿专家
优秀论文
相关链接
摘要
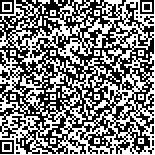
近年来,深度学习算法得到了长足的发展,并开始应用于云检测。但是深度神经网络模型参数众多,依赖大量训练样本,因此理解其泛化性能对于深度学习在不同遥感影像的实际应用具有重要的参考价值。本文以深度语义分割算法DeepLabv3+为例,以一组广泛使用的云标记数据集“L8 Biome”为验证数据,探讨该算法用于云检测时在不同的地表景观、空间分辨率和光谱波段组合的遥感影像上的泛化性能。云标记数据集“L8 Biome”包含96景具有全球代表性的Landsat 8 OLI影像及相应的人工云掩膜,被广泛用于测试云和云阴影检测算法性能。首先,利用Landsat 8 OLI云标记数据集“L8 Biome”,构建不同类型景观、不同空间分辨率、不同波段组合的训练影像集和测试影像集;其次基于不同训练样本集和测试集,评估了DeepLabv3+算法在不同情况下的云检测精度,并与Fmask算法作对比分析。研究结果表明:(1)使用全混合景观类型的训练集训练出来的云检测网络在总体检测精度(92.81%)与稳定度(标准差12.08%)上都优于使用单一景观类型的训练集训练得到的云检测网络,也优于Fmask的总体精度(88.75%)与稳定度(标准差17.34%),说明在构建深度学习算法的训练集时,应该尽可能包含多类型的地表景观;(2)将全混合景观训练集中剔除一类景观的样本(冰/雪景观除外)构建的“混合-1”训练集与全混合景观训练集训练的DeepLabv3+网络的云检测精度也相差不大,说明现有训练样本集已具备较强的景观泛化能力;(3)基于30 m空间分辨率的全混合景观训练样本集训练得到的DeepLabv3+云检测网络在不同分辨率(30 m、60 m、120 m、240 m)的测试集上云检测精度差异不大,都取得较好的效果,说明DeepLabv3+能够泛化应用于不同空间分辨率的遥感影像,相反Fmask直接应用于低分辨率影像时精度明显下降;(4)DeepLabv3+能充分自适应不同波段的信息用于云检测,总体来说更多的光谱波段输入能够提高DeepLabv3+的云检测的精度和稳定度,其中短波红外波段对于DeepLabv3+区分冰/雪与云具有重要价值,而热红外波段对DeepLabv3+云检测网络的性能提升很微小。以上结果说明利用现有数据集“L8 Biome”训练的DeepLabv3+云检测网络能够适用于多种类型的遥感影像,并优于Fmask算法。
Deep learning algorithms have been developed and applied in detecting clouds for satellite imagery in recent years. However, deep neural network models consist of thousands or millions of parameters, thus usually requiring large amounts of training data. Therefore, understanding the generalization ability of deep learning techniques is vital in their application to cloud detection of different types of satellite imagery. Taking DeepLabv3+, a typical deep semantic segmentation algorithm, as an example, this study explored the generalization ability of the algorithm on the cloud detection of satellite imagery with different landscapes, spatial resolutions, and spectral band combinations based on the cloud labeled dataset “L8 Biome.” The “L8 Biome” dataset consists of 96 typical Landsat 8 OLI images and the corresponding manual cloud mask, which has been widely used for evaluating the performance of cloud and cloud shadow detection methods. First, the cloud labeled dataset “L8 Biome” was used to generate different training and test samples with different landscapes, spatial resolutions, and band combinations. Then, the performance of DeepLabv3+ was evaluated based on different training and test sets and compared with that of the typical Function of Mask (Fmask) algorithm. Results show the following: (1) the DeepLabv3+ trained by a fully mixed training set (consisting of imagery captured over all landscapes) has higher overall cloud accuracy (92.81%) and stability (standard deviation 12.08%) than that trained by the training set of imagery captured over a single landscape and performed better than the Fmask algorithm with an overall cloud accuracy of 88.75% and stability of 17.34%, indicating that the training set of the deep learning algorithm should include images captured over various landscape types; (2) the DeepLabv3+ trained by “mixed-1” training sets, which were built by removing the images captured over a single landscape type (except of snow/ice) from the fully mixed training set, achieved comparable accuracies with that trained by a fully mixed training set, indicating that the available training set is diverse enough and has a good generalization ability on imagery captured over different landscapes; (3) the DeepLabv3+ trained by a fully mixed training set with a 30-m resolution achieved similar cloud detection accuracies on the satellite images with different spatial resolutions (i.e., 30, 60, 120, and 240 m), indicating that the trained DeepLabv3+ could be directly applied on satellite imagery with different spatial resolution, whereas the Fmask algorithm performed poorly on the images with coarse resolutions; (4) DeepLabv3+ could explore the effective information from different spectral bands for cloud detection, and more spectral band input can generally improve the overall cloud accuracy and stability of DeepLabv3+. Among all input bands, shortwave infrared bands are greatly helpful for distinguishing snow/ice from clouds, whereas the thermal infrared band marginally improves the cloud detection accuracy for DeepLabv3+. Results indicate that the DeepLabv3+ cloud detection network trained by the “L8 Biome” dataset can be applied to various types of satellite imagery and outperforms the Fmask algorithm.