下载中心
优秀审稿专家
优秀论文
相关链接
摘要
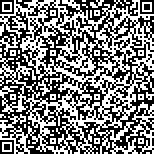
高分辨率遥感图像建筑物分割的实质是构建一个输入图像到分割结果之间的高维强非线性映射模型。然而,建筑物可能遍布整幅遥感图像,则在语义分割过程中,当前像素点可能与非邻域的像素点存在直接关系。为了更加精确地逼近建筑物分割的真实映射模型,克服道路、建筑物错层和阴影的影响,提高分割精度,本文以深度残差神经网络为基础,构建Encoder-Decoder的深度学习架构,自动提取建筑物的特征,学习建立高维强非线性分割模型;同时,通过条件随机场的成对势函数调节当前像素点与其他像素点之间的关联关系,从而构成全连接条件随机场对Encoder-Decoder的分割结果进行调节,提升分割精度。在全连接条件随机场的计算过程中,采用循环神经网络的运行机制来完成均值场的计算,这将条件随机场与深度神经网络有机融合,实现了Encoder-Decoder和全连接条件随机场参数的同步训练。实验结果表明,本文采用的深度神经网络条件随机场方法能有效克服道路、建筑物错层和阴影的影响,提升高分辨率遥感图像中建筑物的分割精度;同时,在一定范围内对多分辨率遥感图像具有较好的泛化能力。
The core of building segmentation in high-resolution remote sensing image is to establish the mapping from an image feature space to a segmentation result with high dimension and strong nonlinearity. In a high-resolution remote sensing image, a building frequently emerges at any location in the entire image, thereby indicating that non-neighborhood pixels may be related to the current semantic segmentation pixel. The segmentation precision and generalization are significantly improved by adopting a Deep Neural Network (DNN) to extract the features and learn the nonlinear mapping in image segmentation. However, the non-neighborhood feature cannot be directly extracted by the DNN. This study presents an encoder-decoder deep learning architecture with ResNet and Conditional Random Field (CRF) for building semantic segmentation in a high-resolution remote sensing image to obtain high segmentation precision and reduce the obstacles from roads, staggered floors, and shadows.
In the DNN, ResNet is used to establish the encoder for automatically extracting the building features, in which ResNet avoids the problems of vanishing and exploding gradient and accelerates the convergence of DNN weights. Before each convolution operation, batch normalization is adopted to normalize the sampling data and reduce the training difficulty of the DNN. Then, transposed convolution is applied to establish the decoder for reconstructing the image while segmenting the buildings. At the end of the DNN, the CRF is used to adjust the raw segmentation produced by the decoder. The value of a unary potential function in the CRF is given by the raw result of the decoder, and the pairwise potential function denotes the feature of pixel pairs in the entire image, which constructs a fully connected CRF (FCCRF). Considering that the calculation of FCCRF is considerable, a mean field algorithm is used to approximate the pairwise potential function value. Thus, convolution is used to obtain the pairwise potential function value, and a high-dimensional Gaussian filter is applied to implement the convolution operation. The mean field algorithm is implemented through an RNN mechanism. Thus, FCCRF becomes a part of the DNN, and the parameters of the CRF are trained with the encoder and decoder simultaneously.
Experiments are conducted to validate the effectiveness of the proposed methodology. The remote sensing image dataset is Inria Aerial Image Labeling Dataset. A total of 4500 samples with 1000×1000×3 pixels are found in each sample, in which their resolution is 0.3 m. The typical kinds of building, such as building with order, single building with complicated roof, and building without order, are segmented through VGG, ResNet, and the proposed methodology (denoted as ResNetCRF), correspondingly. The results show that ResNetCRF overcomes the interruption of roads in which their color features are similar to the building and effectively reduces the disturbance of staggered floors and shadows. Thus, ResNetCRF obtains the optimal segmentation precision. The multi-resolution experiment demonstrates that ResNetCRF has a strong generalization under a limited range of resolution change.
Accurate mapping of building segmentation is established to reduce the disturbance of roads, shadows, and staggered floors by introducing CRFs in the encoder-decoder based on ResNet to segment the building in a high-resolution remote sensing image. In the future work, we will investigate the reduction of FCCRF calculation, overcome the missing segmentation of small buildings, and reduce the segmentation errors of a building whose color feature is similar to the background without a noticeable edge.