下载中心
优秀审稿专家
优秀论文
相关链接
摘要
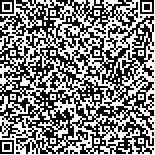
卫星遥感反演海洋内部多时相、大尺度热力结构信息对于了解海洋内部复杂、多维的动力过程有重要意义。本文采用随机森林回归模型,利用卫星遥感观测的海表参量(海表高度异常(SSHA)、海表温度异常(SSTA)、海表盐度异常(SSSA)和海表风场异常(SSWA)),反演不同季节、不同深度层位(1000 m深度以上)的海洋次表层温度异常(STA),并用Argo实测数据作精度验证,采用均方根误差(RMSE)、归一化均方根误差(NRMSE)以及决定系数(R2)评价模型在全球及洋盆尺度上的反演精度。结果显示,全球海洋16个深度层位的平均R2在春、夏、秋、冬四季分别为0.53、0.60、0.54、0.66,NRMSE分别为0.051、0.031、0.043、0.044。随着季节的变化,模型反演性能有所波动。模型在印度洋的反演效果最佳,不同季节、不同深度层位上的平均R2和RMSE分别为0.71和0.18 ℃,而大西洋的反演精度最低,平均R2和RMSE分别为0.46和0.25 ℃。研究表明随机森林模型适用于全球不同季节的STA遥感反演,且在不同洋盆上均有较好的反演效果;不同季节上,上层STA有明显变化信号,空间异质性显著,但300 m以深,STA信号较弱且基本不随季节变化。本研究可为长时序、大尺度海洋内部参量信息遥感反演与重建提供依据,有助于进一步发展深海遥感方法。
Improving ocean interior observation resolution via satellite remote sensing is essential because of the limitation and sparsity of ocean interior observation. Retrieving the multi-temporal and large-scale thermal structure information of the subsurface ocean on the basis of satellite remote sensing is of great importance in understanding the complex and multi-dimensional dynamic processes within the ocean. This task requires a robust model with strong spatiotemporal applicability to provide technical support based on satellite observations.
This study adopts a random forest regression model, namely, an advanced machine learning algorithm, to predict global Subsurface Temperature Anomaly (STA) at different depth levels (upper 1,000 m) in different seasons in 2010 from multisource sea surface parameters (sea surface height anomaly, SSHA; sea surface temperature anomaly, SSTA; sea surface salinity anomaly, SSSA; sea surface wind anomaly, SSWA) based on satellite observations. We use the in-situ Argo data for performance measurement and accuracy validation by combined use of the root mean square error (RMSE), normalized root-mean-square error (NRMSE) and coefficient of determination (R2) at global and ocean basin scales.
For model accuracy, the results show that the average R2 and NRMSE of 16 depth levels are 0.53/0.60/0.54/0.66 and 0.051/0.031/0.043/0.044 for global ocean in spring/summer/autumn/winter. With the evolution of seasons, the model performance promotes first, declines, and then promotes, a trend that may be caused by the El Niño and La Niña phenomena and the transformation between them. The best performance of the model occurs in the Indian Ocean with the average R2 and RMSE of 0.71 and 0.18℃, respectively, whereas accuracy in the Atlantic is the lowest, with average R2 and RMSE of 0.46 and 0.25℃ at different depth levels in different seasons.
This study suggests that the random forest model is suitable for retrieving ocean subsurface temperature anomalies in different seasons and can achieve good performance in different ocean basins. STA has distinctive variation signal in the upper ocean (above 300 m) and spatial heterogeneity is considerable in different seasons. However, in the subsurface and deeper layers (below 300 m), STA variation signal is weak over different seasons. This study can provide a basis for remote sensing estimation of STA and further promote the reconstruction of long-term and large-scale ocean internal parameter information (such as thermohaline structure). It can also help develop the subsurface and deeper ocean remote sensing technique.