下载中心
优秀审稿专家
优秀论文
相关链接
摘要
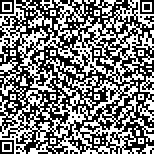
湖泊流域汇水径流过程的模拟预测是一种复杂系统中的时间序列分析问题。模型选择上,现有的机理模型法与辨识模型法各有利弊。同时,现有的模型多采用静态数据驱动模拟,不能有效利用传感网实时观测数据来改善模拟不确定性的问题。本文基于深度循环神经网络技术,提出一种适应动态数据驱动的模式,可融合遥感数据与原位传感器站点数据的DTSM(Dynamic Data Driven Time Series Model)时序模拟预测模型,并在观测值与数值模拟之间建立了一种能动态反馈、自适应调整的模拟框架,解决了传统辨识模型法对时序信息挖掘较弱导致模拟精度较低的问题。通过在鄱阳湖多个子流域入湖径流的案例中验证,显示静态数据驱动模式下,以不同数据源作为输入模拟时,本文DTSM模型的纳希效率系数Ens精度比机理模型提高10个百分点以上;相比静态模式,动态数据驱动模式的模拟精度有进一步提高,尤其是对于静态模式精度较低的流域,提高更为明显。
Performing rainfall-runoff simulation and prediction in a lake basin is a time-series analysis problem in complex systems. Existing mechanistic and identification methods for model selection have advantages and disadvantages. Mechanism model has a clear physical explanation, but it requires professional data for support and the model-solving process is complicated. Identification model is flexible and its solution is simple, but it has difficulty building a universal model and the accuracy of the model is low. Existing simulation models are also based mostly on static data, which cannot effectively use real-time observation data from sensor networks to improve simulation uncertainty. This study aims to improve the problem of traditional identification models being incapable of effectively using timing information, which results in low simulation accuracy. Moreover, it establishes a simulation and simulation framework for dynamic feedback and adaptive adjustment between observation and numerical simulation.
This research proposes a dynamic data-driven model based on deep recurrent neural network, named dynamic data-driven time sequence model, which consists of a multilayered long short-term memory loop body and a fully connected layer. The proposed model incorporates runoff remote sensing rainfall data and ground station observation rainfall data as static data input and recent ground station actual runoff observation data as dynamic data input to simulate catchment runoff process.
Several cases of multiple sub-river runoff into Poyang Lake indicate that in static data-driven mode, with TRMM_3B42_V7 precipitation as input, the ENS accuracy of DTSM is 10 percentage points higher or more than that of the mechanism model. The cases also indicate that in static data-driven mode, with the fusion precipitation from TRMM_3B42_V7 and ground station as input, the ENS accuracy of DTSM is 29 percentage points higher or more than that of the mechanism model. Lastly, the dynamic data-driven model can further improve the accuracy of simulation compared with the static-driven model, and the improvement is substantial in the basin with lower accuracy in static data-driven mode.
The model based on deep recurrent neural network can effectively extract the timing information from the data. The dynamic data-driven model can make adaptive adjustments to improve simulation uncertainty. Based on the above two reasons, the DTSM proposed in this paper can achieve the same or a better simulation accuracy than existing representative mechanism models. At the same time, DTSM is flexible and the solving process is simple.