下载中心
优秀审稿专家
优秀论文
相关链接
摘要
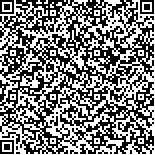
本文利用地基激光雷达实现天然林区近地面点云数据的精细分类和倒木提取。对大兴安岭天然林区的3个倒木样地进行了近地面1.3 m以内点云精细分类和倒木信息提取。为避免点云密度差异和遮挡的形态特征,点云分类时基于自适应临近搜索法计算团块协方差特征值构造3D和2D特征。使用k临近递增的团块协方差特征值得到的线性特征、面状特征和发散状特征构造最大熵函数,用最大熵函数取得最大值时的临近点云计算特征参数,根据递归特征排除法(RFE)筛选重要变量进行随机森林分类。利用自适应kNN特征得到3块研究样地(A、B、C)的分类总体精度分别为93.17%、94.52%、95.16%;固定k临近搜索时,总体精度分别为92.65%、89.09%、92.99%,表明自适应kNN搜索方法使分类精度有一定提高。提取倒木点云去噪处理后进行随机抽样一致圆柱拟合,根据轴线方向进行圆柱的筛选与合并,实现倒木的识别,样地倒木识别率为100%。
Terrestrial Laser Scanning (TLS) can effectively describe complex forest scenes. This study aimed to classify ground point cloud within the height of 1.3 m into ground, vegetation, fallen wood, and standing trunk based on the TLS obtained from fallen wood plots in Daxing'anling. Fallen wood cloud point was segmented and merged. The optimal 3D neighborhood of each individual point was calculated through the Shannon entropy constructed by linearity, planarity, and scattering to avoid the difference in cloud density and the morphological characteristics introduced by occlusion. Shannon entropy could be maximized across the increasing kNN with an interval of 5 points. The optimal neighborhood size was used to compute the covariance eigenvalues for constructing 3D and 2D features. Key features were selected following the recursive feature elimination criteria, and a random forest classification algorithm was used to classify the points. Noise removal approach was applied to the fallen wood points classified by self-adjusting kNN features, and random sample consensus (RANSAC) segmentation was implemented to segment cylinders. Fallen wood cylinders were selected and merged depending on the axis direction less than 12° and the distance less than 0.1 m between each other. The overall classification accuracies of self-adjusting kNN method in plots A, B, and C were 93.17%, 94.52%, and 95.16%, respectively, and corresponding Kappa coefficients were 0.8771, 0.9145, and 0.9242, respectively. The overall accuracies of non-self-adjusting kNN were 92.65%, 89.09%, and 92.99%, and the Kappa coefficients were 0.8684, 0.8909, and 0.9299. Point cloud of plots B and C was classified using the model we trained using plot A. The classification accuracies of plots B and C were 62.38% and 59.80%, and the user precisions of fallen wood point cloud were 79.31% and 48.06%. All fallen woods had the same number as the ground measurement, and the parameters of fallen wood could be estimated roughly. Compared with the non-self-adjusting kNN method, the near-ground point cloud classification accuracy was improved by the self-adjusting kNN point cloud feature. Classification of plots B and C using the training result of plot A suggested that the selected key features in the complex forest could explain the dependent variable well. RANSAC could effectively segment the cylinder and estimate the parameters of the fallen wood. This research is significant for extracting parameters of the existing work. Further ecological research will be considered accordingly.