下载中心
优秀审稿专家
优秀论文
相关链接
摘要
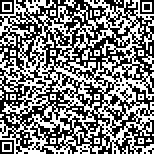
三峡工程蓄水以来,清水下泄,坝下游河段发生了长时间、长距离的沿程冲刷,河流悬浮泥沙浓度发生改变,给沿岸生态系统带来了不利影响。随机森林算法灵活、稳健,已被广泛应用于各类生态环境变量的回归预测分析,但其在水体悬浮泥沙浓度估算方面的能力尚未得到充分认识。基于泥沙站点监测数据和MODIS卫星遥感反射率数据,通过构建随机森林非参数回归预测模型,对三峡工程坝下游宜昌至城陵矶河段在建坝前后14年间(2002年—2015年)各月的悬浮泥沙浓度进行遥感估算。研究表明:(1)基于随机森林的悬浮泥沙浓度估算模型表现较好,模型预测值与实测值间相关性好、预测精度高,优于其他模型(线性回归、支持向量机、人工神经网络模型)。(2)在参与模型构建的MODIS波段变量中,红波段被认为是最重要的预测变量,但不能单独使用它进行预测,悬浮泥沙遥感预测需要多变量共同参与。(3)将悬浮泥沙数据按季节分类所构建的随机森林模型,其平均误差为0.46 mg/L,平均相对均方根误差为12.33%,估算效果最优,能够满足较高精度下悬浮泥沙浓度估算的需求。综上,可以考虑以季节为划分依据,用随机森林回归模型估算悬浮泥沙浓度,并用于后期坝下游河道悬浮泥沙浓度时空反演。
Since 2003 when the Three-Gorge Dam (TGD) was in impoundment, the dam abundantly blocks suspended sediment and cause clear water flowing through the dam, which induces scouring effect on the beds and banks of the Yangtze river below the dam.Furthermore, the altered Suspended Sediment Concentration (SSC) has adversely affected the downstream coastal environment.
In this study, the random forest model was applied for SSC estimation. The model is flexible and robust, and can be used for regression analysis of ecological environment variables. Yet, its ability in estimating SSC in aquatic environment has not been fully understood. On the basis of the monitoring data of SSC and satellite remote sensing reflectance data from 2002 to 2015, this study estimated the SSC in Yichang-Chenglingji downstream reach of the TGD by constructing a non-parametric regression model using random forest.
The results showed that:(1) the random forest model could effectively monitor SSC, and the correlation coefficient and prediction accuracy were significantly improved from those of other models (linear regression, support vector machine, and artificial neural network model).(2) the red band is a suitable predictor for SSC in the random forest model, but cannot be independently used for forecasting. SSC remote sensing prediction requires multivariate co-participation. (3)By using the random forest model, the average root mean square error of the seasonal division was 0.46 mg/L, and the average relative root mean square error was 12.33%. These values met the needs of high-precision SSC estimation.
In conclusion, this study reveals that the season shall be considered as temporal factors to estimate SSC and then prepare for the subsequent SSC spatiotemporal inversion. Which is of great help to reveal the TGD's downstream river sediment evolution, and understand the regional distribution of sediment and sediment variation process in the future.