下载中心
优秀审稿专家
优秀论文
相关链接
摘要
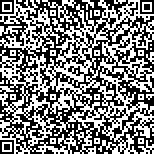
遥感技术具备实时快速、时空连续、广覆盖尺度等独特优势,在全球气候恶化大背景下,利用遥感干旱监测方法相比于传统地面监测手段,能够提供实时、准确、稳定的旱情信息,辅助科学决策。目前常用遥感旱情监测方法大多依赖全域性数学模型建模,假定了旱情模式的空间平稳特性,因而难以准确反映旱情模式的局部差异特征。本文提出利用地理加权回归模型GWR (Geographically Weighted Regression),考虑旱情模式的空间非平稳特性,综合多种遥感地面旱情监测指数,以实现传统全域旱情监测模型的局部优化。以美国大陆为研究区,监测2002年—2011年共10年的旱情状态。研究表明,GWR模型能够提供空间变化的局部最佳估计模型参数,监测结果更加吻合标准美国旱情监测USDM (U.S Drought Monitor)验证数据,且与地面实测值的最高相关系数R达到0.8552,均方根误差RMSE达到0.972,显著优于其他遥感旱情监测模型。GWR模型具备空间非平稳探测优势,实现了旱情模式的局部精细探测,能够显著提升遥感旱情监测精度,具备较好的应用前景。
Remote sensing technology has the unique advantages of real-time, fast and spatio-temporal continuity and wide coverage scale. Under the background of global climate deterioration, drought monitoring methods based on remote sensing can provide more real-time, accurate, and stable drought information and better assist scientific decision making than traditional ground monitoring methods. (Methods) Most of the existing drought monitoring methods based on remote sensing rely on global mathematical models that assume the spatial stability of drought events; hence, an accurate representation of local difference characteristics is difficult to achieve. In the current study, a geographic weighted regression (GWR) model is proposed to optimize the traditional global drought monitoring model by considering the spatial non-stationary characteristics of drought events and synthesizing various remote sensing drought indices. (Results) This study, which was conducted in mainland United States, focused on drought monitoring over a ten-year period (2002-2011). The results indicate that the GWR model can provide the best model parameters for the local estimation of spatial variations. Moreover, the monitoring results are consistent with the standard verification data of the United States Drought Monitor. The highest correlation coefficient R between the GWR model and the measured data is 0.8552. The RMSE is 0.972, which is significantly superior to other remote sensing drought monitoring models. (Conclusion) The GWR model has the advantage of spatial non-stationary detection and can realize local fine detection in drought modeling. Moreover, the GWR model can significantly improve the precision of remote sensing drought monitoring and thus has a good application prospect.