下载中心
优秀审稿专家
优秀论文
相关链接
摘要
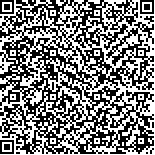
针对云检测在高亮度地表以及雪覆盖区域存在过度检测的问题,设计了一种不依赖热红外波段的增强型多时相云检测EMTCD(Enhanced Multiple Temporal Cloud Detection)算法。首先,利用云的光谱特征建立单时相云检测规则,并基于云、雪的光谱差异构建了增强型云指数ECI(Enhanced Cloud Index),改进了云、雪的区分能力;其次,以同一区域无云影像为参考,基于ECI指数构建了多时相云检测算法,较好地克服了单时相云检测中高亮度地表、雪和云容易混淆的问题,提高了云检测的精度;最后,选择两个典型区域的Landsat-8 OLI影像,对比分析了不同算法的云检测结果。实验结果表明:ECI指数能够有效区分云、雪,EMTCD方法的平均检测精度达到93.2%,高于Fmask(Function of mask)(81.85%)、MTCD(Multi-Temporal Cloud Detection)(66.14%)和Landsat-8地表反射率产品LaSRC(Landsat-8 Surface Reflectance Code)的云检测结果(86.3%)。因此,本文提出的EMTCD云检测算法能够有效地减少高亮度地表和雪的干扰,实现不依赖热红外波段的高精度云检测。
Remote sensing images have been a crucial data source for land cover mapping and other applications. However, optical remote sensing images are frequently contaminated with clouds. Clouds have caused several limitations in remote sensing applications through optical satellite. Although several approaches have been conducted for cloud detection, they still fail to distinguish bright surfaces, snow, and clouds, especially for seasonally snow-covered images. Therefore, we aim to develop a fast and universal cloud detection method, which can accurately detect clouds in complex areas. Considering that many sensors do not have a thermal infrared band, we only use the visible, near infrared, and short-wave infrared bands to detect clouds. The proposed method is expected to be used for a variety of satellite data.
In this study, a multi-temporal cloud detection method was proposed for optical images. Given that snow and clouds have a big difference in short-wave infrared bands, we first developed an Enhanced Cloud Index (ECI) based on the spectral properties of the bands to distinguish them. Then, we proposed an Enhanced Multi-Temporal Cloud Detection (EMTCD) algorithm based on the ECI index and multi-temporal images to extract cloudy pixels. Finally, we tested and compared the algorithm with three classical cloud detection algorithms, namely, Function of mask (Fmask), Cloud Cover Assessment (CCA), and Multi-Temporal Cloud Detection (MTCD) algorithms, to verify the accuracy of the proposed algorithm. Landsat-8 images were used as the data source in this study. Given that many operational cloud detection methods had failed in complex areas, we selected four Landsat-8 OLI scenes in two test areas with typical seasonal snow cover and complicated land covers as our test data. The images were all obtained from 2015. The test areas were the northeast and southwest of China.
Test results indicated that the ECI index can effectively distinguish snow and clouds. The ECI index of snow was higher than that of clouds. The EMTCD method performed well in cloud detection, which had the best cloud detection result with an overall accuracy of 93.2% compared with that of 81.85%, 66.14%, and 86.3% for the classic Fmask, MTCD, and CCA cloud detection methods, respectively.
The ECI index is effective in distinguishing clouds and snow. The EMTCD algorithm can provide a good performance in cloud detection without using the thermal infrared band, even for seasonally snow-covered regions with complicated high brightness ground surface, which is always challenging for traditional cloud detection algorithms. However, the method is developed based on multiple images. Compared with single temporal methods, the proposed method still has some limitations.