下载中心
优秀审稿专家
优秀论文
相关链接
摘要
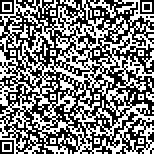
全波形激光雷达的回波中携带了被测目标的距离与特征信息,为了获取这些信息,本文提出了一种回波分解方法。本方法将原始的全波形回波分解为几个独立的高斯脉冲,并得到其函数表达式,从而提取出被测目标的距离等信息。分解过程中,首先,采用可变阈值的经验模态分解滤波法(EMD-soft)对原始波形进行滤波和噪声水平评估;其次,采用一套应对多种波形组成的初始参数估计方法,获取后续拟合所需的初始参数;最后,采用LM(Levenberg-Marquardt)优化算法对回波进行拟合优化,从而获取全波形回波中包含的独立高斯脉冲及其函数表达式。仿真波形的分解实验表明,分解误差在0.1 ns量级,换算成距离误差为15 mm,通过实验室自制的全波形激光雷达实验系统获取的回波的分解实验表明,分解的距离误差小于0.1 m。对比另外两种高斯分解方法对于相同仿真与实验数据的分解结果可以看出,本方法在分解成功率与精度上都有较大的提高。回波分解后的独立高斯脉冲中,除距离外还含有被测目标的反射率、粗糙度、面型等丰富的信息,回波分解方法作为回波分析的基础,将在遥感、测绘等生产与科研领域中发挥非常重要的作用。
The echoes of full-waveform light detection and ranging (LiDAR) contain information on the targets such as distances and properties. To access such information, we demonstrate an echo decomposition method based on soft-thresholding Empirical Mode Decomposition (EMD-soft) filter, Levenberg-Marquardt (LM) optimization, and a method to estimate the initial parameters consisting of a variety of waveforms.
This method decomposes raw full-waveform echoes into independent Gaussian pulses and derives their function expressions. Distances and properties of the targets can be extracted from the parameters of these Gaussian pulses. First, EMD-soft is used to filter the noise of raw echoes and estimate the amount of noise. Then, initial parameters for follow-up optimization are estimated through peak and inflection points of the filtered echoes by a given process. Lastly, after these initial parameters are input, LM optimization is applied to optimize the parameters, and function expressions of Gaussian pulses can be obtained through the parameters. Finally, information on the targets can be accurately extracted.
Simulations and experiments are carried out to evaluate the performance of the proposed method. Simulated waveforms are generated by adding Gaussian white noise into a waveform consisting of several independent Gaussian pulses. Decomposition experiments of these simulated waveforms indicate that the decomposition error of this method is around 0.1 ns, which means 15 mm in ranging error. In actual experiments, a laboratory-built full-waveform LiDAR experimental system is utilized to generate and obtain echoes. Decomposition experiments of these real waveforms also show that the ranging error of this method is less than 0.1 m. Compared with two other Gaussian decomposition methods in decomposing the same echoes mentioned above, this method shows enhanced decomposition success rate and accuracy.
The proposed method realizes high success rate and accuracy decomposition. It can provide reliable data for the subsequent analysis and will play an important role in many fields, such as remote sensing and mapping.
Compared with other decomposition methods, the EMD-soft we used does not have a threshold of filter frequency, that is, it will be effective in any frequency, unlike some other filters that will make mistakes when close to their thresholds. In addition, EMD-soft does not need a given kernel function or parameter, which means it is a more generic method to deal with various LiDAR echoes. LM optimization is one of the most popular algorithms for optimizing LiDAR echoes, but requiring high-accuracy initial parameters is a big problem for the algorithm. Sometimes if the initial parameters do not have enough accuracy, the algorithm may converge to a local minimum instead of a global minimum and lead to a wrong fitting. Thus, in this method, a special process is realized in the section on evaluating initial parameters and enhancing the optimization effect from another way.
Echo decomposition is the foundation of echo analysis. We will try to extract more properties of targets from the decomposed waveforms and build the functional relationship of these properties with the parameters of waveforms in future work.