下载中心
优秀审稿专家
优秀论文
相关链接
摘要
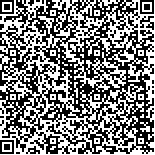
滑坡提取是获取滑坡灾情信息的首要环节。针对背景复杂的震后灾区,为了更加有效地增强裸地信息并降低土壤背景差异对NDVI植土分离能力的影响,利用NDVI进行滑坡提取时结合纹理特征分析和比辐射率估计来提高滑坡提取的精度。首先,根据纹理特征差异将主导背景因子不同的区域划分为独立的处理单元,以避免全局滑坡提取所出现的过提取或欠提取现象;然后,利用NDVI估算比辐射率,以增强裸地信息;最后,通过阈值分割实现更高精度的滑坡提取。围绕5·12大地震后的汶川县城及其周边区域,采用Landsat 5 TM和Terra ASTER数据展开实验,该方法的滑坡提取结果与人工提取结果吻合度较好,Kappa系数分别为0.8531和0.9271,优于基于NDVI的全局阈值分割法和其他一些典型的监督分类法。实验结果表明,在背景复杂的灾区环境下,结合纹理特征分析和比辐射率估计的滑坡提取方法能明显降低漏检率和错检率,进而有效提高滑坡提取的精度,且对中等分辨率的不同遥感数据源的适用性较强。
Landslides are the most common geological disasters that result from large seismic activities and heavy rainfall in mountainous areas. They are destructive events that occur suddenly and have a wide distribution range. Landslide extraction is the primary factor in collecting destruction information and plays a key role in disaster prevention and emergency rescue. This paper presents a method that combines texture analysis and emissivity estimation to extract landslides from different backgrounds. Many researchers have focused on landslide extraction and recognition, and they proposed methods based on the ability of the Normalized Difference Vegetation Index (NDVI) to separate vegetation. However, although NDVI easily extracts landslides from high vegetation areas, it is affected by soil interference in sparse vegetation areas. Thus, extracting landslides is difficult. An NDVI-based method is proposed for landslide extraction in a complicated environment and to make NDVI effective in sparse vegetation zones.
In accordance with the research area and remote sensing data, this method consists of four steps on the assumption that image preprocessing has been completed. First, the NDVI image is calculated by using near-infrared and red bands; this approach shows that some local regions have a sharp contrast, whereas others have a weak contrast. Second, texture analysis is conducted to divide the research area into several blocks according to this contrast distribution. NDVI mean and near-infrared Angular Second Moment (ASM) based on Gray-Level Co-occurrence Matrix (GLCM) could be selected as the texture feature to segment zones easily by providing simple thresholds. A continual large vegetation area is outstanding in the Mean feature, and a mountainous landslide area is rough in the ASM feature. The remaining area, except the first two, varies in the ASM feature related to soil components. Thus, the combination of Mean and ASM features facilitates texture analysis. Third, emissivity is estimated in different blocks based on the NDVI image. In this section, the NDVIs of pure soil and pure vegetation from statistics of the NDVI image in different blocks are critical parameters in calculating the Percentage of Vegetation (PV), thereby obtaining the emissivity. In a limited area, emissivity contrasts within different objects, especially for soil and vegetation, thereby making it more suitable than NDVI for landslide extraction. Finally, the landslides are extracted through emissivity threshold segmentation technology with an appropriate threshold manually.
Landsat 5 TM and Terra ASTER data are tested by this method, and the result is favorable given that it is more consistent with that of artificial landslide extraction than that of the other methods, such as maximum likelihood supervised classification, neural net supervised classification, support vector machine-based supervised classification, and NDVI global threshold segmentation. For objective and quantitative evaluation, the result of artificial extraction is considered as the ground-truth data to calculate the confusion matrix of other results, with the Kappa coefficient being used to demonstrate the performance of each method. As a result, the method described in this article achieves a high Kappa coefficient, namely, 0.8531 (TM) and 0.9271 (ASTER). By contrast, maximum likelihood classification achieves 0.7634 (TM), neural net classification achieves 0.66 (TM), SVM-based classification achieves 0.6896 (TM), NDVI global threshold (0.3) segmentation achieves 0.622 (TM), and NDVI global threshold (0.5) segmentation achieves 0.7487% (TM). Evidently, this method can effectively eliminate omission and misclassification. With the increase in the resolution of remote sensing data, ASTER (15 m) provides a better result compared with TM (30 m), thereby showing that this method does not rely on data sources, and a high resolution contributes to a good result.
Comparative analysis between different methods and data sources indicates the following results: The NDVI mean and near-infrared ASM are good texture features for separating different background environment blocks, especially with soil as the leading factor. Emissivity reflects the spatial difference in objects, although it is estimated by NDVI. The estimated emissivity not only separates vegetation from NDVI but also increases the difference between soils, thereby resulting in good landslide extraction. The advantages of texture analysis and emissivity estimation enhance landslide extraction in complicated research areas. Finally, the threshold selection problem in NDVI global threshold segmentation method is solved, and the sample selection and learning process in supervised classification are avoided. This method is designed for a medium resolution that ranges between 10 m to 50 m; thus, this method can be used with other data sources with a similar resolution, such as Landsat 5 TM and Terra ASTER. We proposed an effective method for landslide extraction for medium resolution. To handle high-resolution remote sensing data, this method will be combined with object-oriented methods in follow-up studies for accurate landslide extraction. The proposed method has a strong dependency on manual threshold selection; this dependency is inconvenient in the auto-extraction process. Therefore, self-adaptive threshold extraction is another challenge in future work.