下载中心
优秀审稿专家
优秀论文
相关链接
摘要
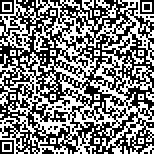
基于机载激光雷达LiDAR(Light Detection and Ranging)数据识别震后建筑物震害,其前提是快速准确地提取建筑物点云。通过分析地震灾区机载激光雷达点云中提取建筑物点云的诸多难点,已有的方法难以达到预期效果,因此提出融合同机航空影像数据的方法,实现了震后灾区建筑物点云的获取。该方法首先在数据预处理的基础上,利用布料模拟滤波CSF(Cloth Simulation Filtering)算法进行点云滤波,得到地面点云和非地面点云(主要是建筑物、植被和车辆行人等),并将航空影像红波段光谱信息赋予非地面点云;然后基于灰度直方图阈值分割的方法剔除植被点;最后对剩余激光脚点利用具有噪声的基于密度的空间聚类DBSCAN(Density-Based Spatial Clustering of Applications with Noise)算法进行聚类提取最终的建筑物点,并与参考建筑物点比对,进行精度验证,得到建筑物点云提取的漏检概率、虚警概率分别为15.61%、7.52%,总体精度可达84.39%。结果表明,在一定精度要求范围内,该方法能有效实现地震灾区建筑物点云的提取,可为震后机载LiDAR建筑物点云提取提供技术参考和方法借鉴,为建筑物震害识别做好基础工作。
关键词:
机载LiDAR 航空影像 地震灾区 建筑物点云 DBSCAN聚类算法Devastating earthquakes are one of the most serious natural disasters that affect mankind. Although humans cannot predict or prevent earthquakes, we can change the way we respond to them. Earthquake-induced building damage is one of the main causes of casualties and property loss. Extracting the information of post-earthquake buildings quickly and accurately is crucial for identifying earthquake-induced building damage and initiating effective rescue of survivors. The geographical environment is complex in the seismic field, and remote sensing technology is a potential alternative to capturing post-event scene data. Numerous studies have focused on researching intact buildings. The identification of building seismic damage based on airborne Light Detection and Ranging (LiDAR) is studied only by using object-oriented analysis, and few papers use the characteristics of building points. This paper proposes a method that extracts building points in the earthquake zone from airborne LiDAR point cloud data.
This paper treats the fast and reliable extraction of the information of a post-earthquake building as a challenging task in which many difficulties arise because of airborne LiDAR point cloud data, and pre-existing methods cannot easily achieve the desired effect. Therefore, this paper presents a method that combines aerial images and LiDAR data to extract post-event building points in the affected quake areas. The approach consists of several steps. We first extract terrain points from the LiDAR point cloud by using the cloth simulation filtering algorithm, and off-terrain points are removed. Then, we manually collect training samples of building and vegetation points and identify the red band as the best spectral characteristic information to maintain two isolated points. This procedure is based on the minimum probability of total error. Afterwards, we assign the gray value of the aerial image red band to the terrain points (which mainly include buildings, vegetation, pedestrians, and vehicle). The vegetation points are eliminated from off-terrain points by using gray-level histogram threshold segmentation. We used the density-based spatial clustering of applications with the noise (DBSCAN) algorithm to extract the final building points from the rest of the laser footprints, which contain discrete noise points.
We can obtain intact and destructive building points from the LiDAR point cloud by using techniques such as point cloud filtering and threshold segmentation based on the gray intensity of the red band. However, some low-density noise points on the road, such as vehicles, pedestrians, and vegetation, can be obtained. The high-density building points remain nearly constant, and discrete low-density noise points are removed effectively by using the DBSCAN algorithm.
The extracted point cloud of a building is compared with reference points to quantitatively verify the extraction accuracy. The false-dismissal probability and false-alarm probability are 15.61% and 7.52%, respectively. The two main causes of undetected error are as follows: (1) The damaged building points, which have a similar height as the ground points, are filtered when the point cloud filter is executed. (2) The building points in the shadow of tall buildings or trees are divided into vegetation points during threshold segmentation. False-alarm error is due to crowded vehicle points in local areas, and these points cannot be removed by using the DBSCAN clustering algorithm. The two errors are small, and the total extraction accuracy is as high as 84.39%, thereby indicating that the extraction of building points in quake-hit regions satisfies requirements in some bounds of precision with the application of the aforementioned method.
Results show that the suggested method can effectively extract building points in the earthquake zone within a certain accuracy requirement and provide technical reference for the extraction of post-earthquake intact or destroyed buildings by using airborne LiDAR point cloud data. The proposed approach could establish the foundation for identifying seismic building disasters and completing post-earthquake building damage assessment.
With the development of laser radar technology, an airborne LiDAR remote sensing system can receive dense point cloud data while acquiring multispectral or hyperspectral data. Hence, we will improve the ability of the proposed approach to distinguish between vegetation and buildings by using threshold segmentation based on normalized difference vegetation index or other band combinations to further improve the accuracy of building point extraction in the seismic field.