下载中心
优秀审稿专家
优秀论文
相关链接
摘要
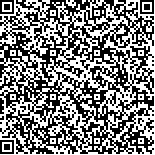
大范围、长时间和高精度农作物空间分布基础农业科学数据的准确获取对资源、环境、生态、气候变化和国家粮食安全等问题研究具有重要现实意义和科学意义。本文针对传统阈值法农作物识别过程中阈值设置存在灵巧性差和自动化程度低等弱点,以中国粮食主产区黄淮海平原内河北省衡水市景县为典型实验区,首次将全局优化算法应用于阈值模型中阈值优化选取,开展了利用全局优化算法改进基于阈值检测的农作物分布制图方法创新研究。以冬小麦为研究对象,国产高分一号(GF-1)为主要遥感数据源,在作物面积统计数据为总量控制参考标准和全局参数优化的复合型混合演化算法SCE-UA (Shuffled Complex Evolution-University of Arizona)支持下,提出利用时序NDVI数据开展阈值模型阈值参数自动优化的冬小麦空间分布制图方法。最终,获得实验区冬小麦阈值模型最优参数,并利用优化后的阈值参数对冬小麦空间分布进行提取。通过地面验证表明,利用本研究所提方法获取的冬小麦识别结果分类精度均达到较高水平。其中冬小麦识别结果总量精度达到了99.99%,证明本研究所提阈值模型参数优化方法冬小麦提取分类结果总量控制效果良好;同时,与传统的阈值法、最大似然和支持向量机等分类方法相比,本研究所提阈值模型参数优化法区域冬小麦作物分类总体精度和Kappa系数分别都有所提高,其中,总体精度分别提高4.55%、2.43%和0.15%,Kappa系数分别提高0.12、0.06和0.01,这体现出SCE-UA全局优化算法对提高阈值模型冬小麦空间分布识别精度具有一定优势。以上研究结果证明了利用本研究所提基于作物面积统计数据总量控制以及SCE-UA全局优化算法支持下阈值模型参数优化作物分布制图方法的有效性和可行性,可获得高精度冬小麦作物空间分布制图结果,这对提高中国冬小麦空间分布制图精度和自动化水平具有一定意义,也可为农作物面积农业统计数据降尺度恢复重建和大范围区域作物空间分布制图研究提供一定技术参考。
Using remote sensing technology to accurately obtain the spatial distribution information of crops in a large region is critical in improving the research level of resources and environment, strengthening the reaction capability of climate change, and ensuring national food security. Threshold method is a common method used to extract crop spatial distribution information based on time-series NDVI remote sensing data. However, several subjectivities and uncertainties were observed in traditional threshold methods when the threshold values were determined by humans, which hindered the improvement of threshold method identification accuracy. Considerable studies on determining the significant threshold value have been conducted in recent years. However, the accuracy and automation should be improved when the threshold values are determined.
Considering the above problems, Jingxian County of Hengshui City in Hebei Province, which is the main grain producing region in China, was selected as the typical experimental area. A global optimization algorithm was applied to select the threshold value in the threshold model, and a crop distribution mapping method using global optimization algorithms based on threshold detection was developed. In this study, winter wheat was selected as the research crop, and domestic Gaofen-1 satellite images that cover the entire winter wheat growing season were used as major data source. A new spatial distribution mapping method for winter wheat based on time-series NDVI data for the automatic optimization of threshold model parameters was proposed in this study by using the global optimization algorithm Shuffled Complex Evolution-University of Arizona (SCE-UA) and crop area statistics as the total quantity constraint. First, the NDVI curves of winter wheat growth season were established, and the key phenological characteristics of winter wheat were extracted based on crop phenological and training sample data. Second, the general threshold model of winter wheat spatial distribution extraction was established, and the threshold parameters to be optimized were determined based on the seasonal variation and characteristics of time-series winter wheat NDVI at key phenological stages (such as seeding, tillering, jointing, heading, and maturation stages). Third, the optimal parameters of winter wheat spatial distribution model were obtained by the global optimization algorithm SCE-UA by using the crop planting area statistics at county level as total quantity constraint data and objective function. Finally, the optimal parameters of winter wheat threshold model were obtained in the study region, the spatial distribution of winter wheat was extracted by using the optimized threshold parameters, and the winter wheat distribution extraction results were validated by the ground verification samples.
The final validation results showed that the accuracy of winter wheat identification results reached 99.99%, which proved that proposed threshold parameter optimization method had a good total quantity constraint effect and other classification accuracies had reached a high level. The overall accuracy and kappa coefficient were 97.03% and 0.94, respectively. Compared with the traditional threshold, support vector machine, and maximum likelihood methods, the overall classification accuracy of the proposed method increased by 4.55%, 2.43%, and 0.15%, respectively. The kappa coefficient of the proposed method increased by 0.12, 0.06, and 0.01, respectively. The above performances indicated that the crop distribution mapping optimization method based on the threshold model parameters of statistical data total quantity constraint and global optimization algorithms was effective and feasible to accurately obtain the spatial distribution mapping results of crops in a large region. This study can serve as basis to improve the accuracy and automation level of crop spatial distribution identification in China and provide several technical support and recommendations for other studies on crop spatial distribution extraction and crop mapping under complex planting pattern at large scale.