下载中心
优秀审稿专家
优秀论文
相关链接
摘要
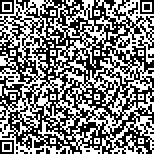
高分辨率遥感图像场景分类方法主要涉及两个环节:特征提取以及特征分类,分类器的设计已经相对成熟,当前工作的重点是特征提取策略的研究。为了进一步推动特征提取策略的研究,将特征提取策略对高分辨率遥感图像场景分类性能的影响进行了定性和定量评估。首先,回顾了高分辨率遥感图像场景分类的发展历程;然后,对现有高分辨率遥感图像场景分类方法的特征提取策略进行分类总结,并从理论上将各类特征提取策略对场景分类性能的影响进行定性评估;最后,在3个规模较大的数据集上对多种特征提取策略进行实验对比,将不同特征提取策略对场景分类性能的影响和各数据集的复杂度进行定量评估。
Remote sensing image scene classification aims to tag remote sensing images with semantic categories according to the content of the image and is important in disaster monitoring, environmental detection, and urban planning. Scene classification results can provide valuable information about object recognition and image retrieval and can effectively improve the performance of image interpretation. The general process of remote sensing image scene classification mainly consists of feature extraction and scene classification based on image features. Given that the design of classifiers is relatively mature, this work focuses on feature extraction strategy. The influence of various strategies on the performance of scene classification is short of unified evaluation, which limits its development. The effect of various feature extraction strategies on the performance of high-resolution remote sensing image scene classification is evaluated in this study.
In the second section of this paper, existing feature extraction strategies are divided into two categories:(1) hand-designed and (2) data-driven feature extraction. Hand-designed features, such as Color Histograms (CH) and Scale Invariant Feature Transform (SIFT), provide the primary description of images and are presented in the early period. Further abstract description of the images is introduced by coding of hand-designed features, such as Bag of Visual Words (BoVW) and has higher classification accuracy than hand-designed features. However, these feature extraction strategies generally suffer from poor generalization capability due to specific requirements for designing. Furthermore, hand-designed features require significant domain knowledge. By contrast, data-driven features can directly learn powerful features from a large number of sample images and are generally divided into shallow and deep learning features. Shallow learning feature extraction mainly involves Principal Component Analysis (PCA), Independent Component Analysis (ICA), and sparse coding algorithms. Typical deep learning feature extraction strategies include stacked autoencoder (SAE), Deep Belief Network (DBN), and Convolutional Neural Network (CNN). Compared with deep learning models, shallow learning models can be regarded as a neural network with a single hidden layer and thus cannot capture high-level semantic features. The superiority of deep learning features is obvious when dealing with complex scene classification. Furthermore, CNN-based features exhibit improved performance compared with SAE-and DBN-based features because the one-dimensional structure of SAE and DBN destroys the spatial information of images.
In the third section of this paper, 29 feature descriptors are quantitatively compared in UC Merced, AID, and NWPU RESISC-45 datasets and eight combinations of feature descriptors are quantitatively compared in the NWPU RESISC-45 dataset. The effect of different feature extraction strategies on the performance of scene classification and the complexity of each dataset are evaluated through quantitative comparison. The experimental results are as follows. (1) The classification accuracy and stability of hand-designed features is poor, however the efficiency of most features is satisfactory and can attain better performance by combining with other types of features. (2) Among all feature extraction strategies, the coding of hand-designed features possesses moderate levels of classification accuracy, efficiency, and stability. (3) The classification accuracy and stability of data-driven features are best, but most of them have low efficiency. (4) AlexNet, a deep learning model with few layers, exhibits the best comprehensive performance and is suitable for occasions that require high classification accuracy, efficiency, and stability. (5) Some scene classes belonging to land use type are easy to be confused because of similar landmark buildings or sites. Moreover, some scene classes belonging to land cover type are easy to be confused because of their similar geomorphologic features. (6) The recently proposed NWPU RESISC-45 dataset is more complex than the other datasets and is more challenging for scene classification algorithms.
Finally, the summary and conclusion of this paper are presented, and the discussion of future development is provided. On the one hand, combining prior knowledge introduced by hand-designed features with the CNN model may be one of the future development directions. On the other hand, introducing Generative Adversarial Networks (GAN) into CNN training may be a research hotspot in the future. In addition, remote sensing parameters, such as NDVI and NDWI, and multi-spectral information can be integrated with current feature extraction strategies for practical applications.