下载中心
优秀审稿专家
优秀论文
相关链接
摘要
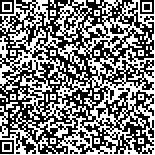
中国是世界上人工林面积最大的国家,实时、定量、精确地获取人工林林分特征对于人工林资源监测、管理以及全球碳循环具有重要意义。以北亚热带沿海平原人工林为研究对象,借助机载激光雷达LiDAR(Light Detection And Ranging)点云数据并结合地面实测的55个样地来反演人工林林分特征。首先,构建冠层高度分布剖面CHD(Canopy Height Distribution)和枝叶剖面FP(Foliage Profile);然后,通过Weibull函数分别对CHD和FP进行拟合并提取Weibull参数作为特征变量(第1组);同时,还直接基于点云提取了LiDAR高度变量HRM(Height-Related Metrics)和冠层密度变量DRM(Density-Related Metrics) (第2组);最后,结合地面实测数据和两组特征变量构建了多元回归模型用于预测各林分特征(即林分密度、平均胸径、胸高断面积、Lorey's树高、蓄积量和地上生物量)。结果表明:(1)与只使用基于点云的特征变量(即第2组)相比,结合点云特征变量(第2组)和冠层垂直结构剖面特征变量(第1组)的各林分特征预测精度均有所提升(ΔAdjusted R2=0-0.13, ΔrRMSE=0.08-3.65%);(2)对各林分特征预测的结果中,Lorey's树高(Adjusted R2=0.85, rRMSE=7.66%)和蓄积量(Adjusted R2=0.84, rRMSE=14.27%)的预测精度最高,地上生物量(Adjusted R2=0.78, rRMSE=14.15%)、胸高断面积(Adjusted R2=0.73, rRMSE=14.70%)和平均胸径(Adjusted R2=0.64, rRMSE=15.05%)次之,林分密度(Adjusted R2=0.58, rRMSE=26.16%)的预测精度最低;(3)Weibull函数较准确地反映了亚热带人工林垂直冠层结构,可以有效提高林分特征反演精度。
China is a country with the largest plantation area in the world. Timely, quantitatively, and accurately acquiring planted forest stand characteristics is the key to monitoring and managing forest plantation, which contributes to the global carbon cycle.
This study was conducted at a north subtropical coastal plain forest plantation to estimate forest stand characteristics using light detection and ranging (LiDAR) point clouds combined with 55 field-measured data. First, Canopy Height Distribution (CHD) and Foliage Profile (FP) were extracted and used to obtain Weibull parameters by fitting a Weibull density function to the profiles (Suite 1). Second, Height-Related Metrics (HRM) and Density-Related Metrics (DRM) (Suite 2) were directly extracted from the LiDAR point clouds. Finally, multiple regression models were constructed to predict the stand characteristics (i.e., stand density, diameter of breast height (DBH), basal area, Lorey's tree height, volume, and aboveground biomass) on the basis of the field-measured data and two suites of metrics.
Results showed that (1) the accuracies of the forest stand characteristics that use point cloud (Suite 2) and canopy vertical profile metrics simultaneously (Suite 1) were relatively higher than that only using point cloud metrics (Suite 2) (ΔAdjusted R2=0-0.13, ΔrRMSE=0.08%-3.65%). (2) For all the forest stand characteristic estimations, the models of Lorey's tree height (Adjusted R2=0.85, rRMSE=7.66%) and volume (Adjusted R2=0.84, rRMSE=14.27%) demonstrated the highest accuracies, followed by the aboveground biomass (Adjusted R2=0.78, rRMSE=14.15%), basal area (Adjusted R2=0.73, rRMSE=14.70%), and mean DBH (Adjusted R2=0.64, rRMSE=15.05%). Stand density exhibited the lowest accuracies (Adjusted R2=0.58, rRMSE=26.16%). (3) The Weibull function was appropriate for describing the vertical stand canopy structure of planted forests. The Weibull metrics can be favorable in effectively improving the estimation accuracy of the forest stand characteristics.
This study provided an effective approach in estimating the forest stand characteristics that use the LiDAR point clouds by combining vertical profile and point cloud metrics. This study also proved that the vertical profile metrics can help in increasing the accuracy of the predictive models and provide the mechanism for interpreting the forest stand vertical structure.