下载中心
优秀审稿专家
优秀论文
相关链接
摘要
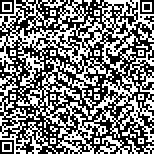
传统的基于鲁棒主成分分析的高光谱异常探测模型中,稀疏异常矩阵假设为非低秩且其非零元素满足随机分布条件。这导致稀疏矩阵的非零元素影响低秩背景矩阵的估计,进而制约背景信息和异常信息的有效分离。提出列式鲁棒主成分分析的异常探测方法,改进异常矩阵为列稀疏条件来解决上述问题。该方法分解高光谱影像2维矩阵为低秩背景矩阵,列稀疏异常矩阵和噪声矩阵,松弛目标方程为凸优化问题,并采用非精确增强拉格朗日乘子算法来求解得到列稀疏异常矩阵的最优估计。最后,对稀疏异常矩阵中所有列的L2范数值进行阈值分割来探测得到异常像元。利用两个高光谱影像数据集,对比5种主流的异常探测方法来验证提出方法的有效性。实验结果表明,列式鲁棒主成分分析方法优于包括传统鲁棒主成分分析模型在内的5种异常探测方法,且计算效率适中。
关键词:
高光谱遥感 异常探测 鲁棒主成分分析 列稀疏 非精确增强拉格朗日乘子Hyperspectral imagery (HSI) collects the detailed spectral response of ground objects on the Earth's surface by using hundreds of narrow bands and presents a great potential for use in detecting anomalies (i.e., small and low-probability ground objects) from the main background. Sparsity theory has recently attracted increasing interest because of intelligent processing in the hyperspectral field. Many sparsity-based anomaly detection methods have been proposed in literature, and robust principal component analysis (RPCA)-based detectors are typical examples. However, regular RPCA-based anomaly detectors show that the sparse anomaly matrix should not be of low rank, and its nonzero entries should be randomly scattered in the matrix. This condition negatively affects the estimation of a low-rank background matrix and seriously impacts the effective separation between the background and anomalies in the image scene. Moreover, RPCA-based approaches involve many iteration procedures of primal variables, which leads to high computational complexity. Therefore, this study proposed column-wise RPCA (CWRPCA) to resolve these problems and improve the detection results.
CWRPCA assumes that background information exists in low-dimensional randomized column subspace and possesses low-rank properties and that the anomalies are sparse and do not lie in the column subspace of the background. CWRPCA aims to decompose the HSI data matrix into the sum of a low-rank background matrix, a column-sparse anomaly matrix with small portions of nonzero columns, and a noise matrix. In this study, when the column subspace of the background was determined, anomalies were estimated from the nonzero columns of the anomaly matrix. The problem of determining the background and anomaly matrices was formulated into a convex optimization program in (2). The inexact augmented Lagrange multiplier algorithm was implemented to optimize the objective function. This algorithm introduces two auxiliary variables into the objective function and iteratively updates primal variables by fixing other variables. The low-rank background and sparse anomaly matrices were obtained when the iteration procedure terminated. The detection result was achieved by segmenting the L2 norms of column vectors in the anomaly matrix.
Two HSI datasets were used to verify the detection performance of CWRPCA. The Receiver Operating Characteristic (ROC) curve and Area Under Curve (AUC) were utilized to evaluate detection performance. ROC describes the probabilities of detection and false alarm. AUC quantifies the area under the ROC curve and shows how far the ROC curve is from the baseline. The detection results of the proposed method were compared with those of five state-of-the-art anomaly detection methods, namely, low-rank and sparse matrix decomposition-based anomaly detection method, global Reed-Xiaoli method, dual window-based eigen separation transform, collaborative representation-based detector, and low-rank and sparse representation. Experimental results showed that the proposed CWRPCA outperformed the five state-of-the-art anomaly detection methods in temrs of ROC curve and AUC with a moderate computation cost.CWRPCA is better in detecting anomalies than other methods and can be a good alternative for hyperspectral anomaly detection.