下载中心
优秀审稿专家
优秀论文
相关链接
摘要
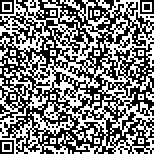
为了解决高光谱遥感影像分类中单一尺度特征无法有效表达地物类间差异和区分地物边界的不足,提高影像分类精度和改善分类目视解译效果,提出了采用引导滤波提取多尺度的空间特征的方法。首先,利用主成分分析对高光谱影像进行降维,移除噪声并突出主要特征;然后,将第1主成分作为引导影像,将包含信息量最多的若干主成分分别作为输入影像,应用依次增加的滤波半径分别进行引导滤波处理提取多个尺度的特征,获得影像不同尺度的结构信息;最后,将多尺度特征输入分类器中进行影像监督分类。采用仿真数据和帕维亚大学(Pavia University)、帕维亚城区(Pavia Centre)等3幅高光谱实验数据,提取了基于引导滤波的多尺度特征、多尺度形态特征和多尺度纹理特征,输入到支持向量机、随机森林和K近邻分类器中,进行了实验。实验结果表明:采用支持向量机分类Pavia University数据,相对于采用多尺度形态特征的分类结果,引导滤波特征的总体精度提高了6.5%;Pavia Centre和Salinas两幅影像最高分类精度均由引导滤波特征实现,分别达到98.51%和98.39%。实验证实基于引导滤波提取的多尺度特征能有效地描述地物结构,进而获得更高的分类精度和改善目视解译效果。
Features extracted from a single scale cannot effectively express differences among land objects and recognize object boundaries.Thus, hyperspectral image classification suffers from low classification accuracy and the "pepper-and-salt" phenomenon. In this context, we propose a set of multi-scale spatial features that is based on guided filtering to improve the performance of image classification in quantitative accuracy and visual interpretation.
The structure-transferring property of the guided filtering is investigated to appropriately represent land objects with different sizes. The proposed feature extraction and classification algorithm consists of three steps. First, Principal Component Analysis (PCA) is used to reduce the dimensionality of hyperspectral images and remove noise. Second, the first several principal components that have the most amount of information are guided by the first principal component to obtain filtered features. Multi-scale features are extracted by guided filtering with increasing radii, which can represent structures of different land cover types. Finally, the feature set is fed into classifiers for image classification.
We operated experiments on one synthetic and three hyperspectral datasets, namely, Pavia University, Pavia Center, and Salinas. Multiple multi-scale features, including multi-scale features based on guided filtering, multi-scale morphological, and multi-scale texture features, were extracted. Three advanced classifiers, namely, Support Vector Machine(SVM), Random Forest(RF), and K-Nearest Neighbor(KNN), were considered for comparison. The results of the experiments conducted on the synthetic data set showed that the proposed feature extraction method could better smooth the inner pixels of land objects while preserving object boundaries compared with multi-scale morphological features. Compared with the combination of multi-scale morphological features and SVM, the combination of multi-scale guided filtering features and SVM achieved a 6.5% increment in overall accuracy on the Pavia University dataset. The highest classification accuracies for the Pavia Centre and Salinas images were 98.51% and 98.39%, respectively.These resultswere achieved by the proposed multi-scale guided filtering method.
A single scale cannot effectively represent the spatial information of land objects. Thus, multi-scale features are extracted using guided filtering. These features can preserve the spatial structures transferred from the guidance image and smooth the details of the input image due to the structure-transferring property of guided filtering. Land objects with various scales can also be effectively described by these multi-scale filtering features by setting different filtering window sizes. The quantitative results and visual inspection showthat multi-scale filtering features can effectively describe the structures of land objects in comparison with morphological and texture features and thus result in high classification accuracy and better visual quality. The proposed feature is suitable for analyzing land scenes with complicated structures, such as urban areas.