下载中心
优秀审稿专家
优秀论文
相关链接
摘要
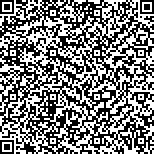
2. 江苏省地理信息资源开发与利用协同创新中心, 南京 210023
空间聚类应当同时满足空间位置邻近和属性相似,在此背景下,为满足空间邻近实体之间趋势性和不均匀性的属性聚类需求,提出一种基于图论和信息熵的空间聚类算法。该算法主要是在Delaunay三角网空间位置聚类基础上,通过引入信息熵,采用多元相似性度量方法以解决二元关系在属性聚类中的缺陷,同时基于“等概率最大熵”原则提出了一种局部参数度量方法,用于表达邻近目标间属性分布的局部变化信息。将本文方法与多约束聚类方法和DDBSC聚类方法进行对比分析,结果表明:(1)在属性空间分布不均的情况下,本文方法的聚类精度要高于多约束方法和DDBSC方法,尤其是当属性空间分布不均程度不断扩大时,DDBSC和多约束算法会将空间簇内的实体误判为噪声;(2)在对异常值的敏感性问题上,3类方法都能识别出异常值的位置,但DDBSC和多约束算法对异常值具有一定的敏感性,聚类结果会掩盖属性分布的趋势性,本文方法受异常值影响很小。通过模拟实验和实际算例可以发现,在保证空间邻近的基础上本文方法具有如下优势:第一,能反映实体属性在空间分布中的趋势性特征;第二,能满足属性空间分布不均匀;第三,对异常值具有良好的稳健性。
关键词:
空间聚类 Delaunay三角网 信息熵 趋势性 不均匀性Spatial clustering is important for spatial data mining and spatial analysis. Spatial objects in the same cluster should be similar in the spatial and attribute domains. Tendency and heterogeneity are important characteristics of geographic phenomena. Currently, most spatial clustering algorithms only consider either tendency or heterogeneity, failing to obtain satisfied clustering results. To overcome these limitations, a spatial clustering method based on graph theory and information entropy is developed in this work.
The proposed algorithm involves two main steps: construct spatial proximity relationships and cluster spatial objects with similar attributes. Delaunay triangulation with edge length constraints is first employed to construct spatial proximity relationships among objects. To obtain satisfactory results in spatial clustering with attribute similarity, the information entropy is introduced to overcome the defects of similarity measure with binary relation, which can reflect the clustering tendency of geographical phenomena. Furthermore, a local parameter measurement method based on the principle of “equal probability maximum entropy” is designed to adapt to the local change information of attribute distribution.
The performance of the proposed algorithm was evaluated experimentally by comparing the leading state-of-the-art alternatives: DDBSC and multi-constraint algorithms. Results showed that our method outperformed the two other algorithms as attributes are unevenly distributed in space. The sensitivity analysis of these algorithms showed that our method was the least sensitive to outliers.
The effectiveness and practicability of the proposed algorithm were validated using simulated and real spatial datasets. Two experiments were performed to illustrate the three advantages of our algorithm: (1) It can reflect the tendency of the entity attribute in the spatial distribution. (2) It can meet the requirement that attributes are unevenly distributed in space. (3) It can discover clusters with arbitrary shape and is robust to outliers.