下载中心
优秀审稿专家
优秀论文
相关链接
摘要
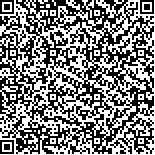
基于表层卫星遥感观测的中深层海洋遥感对于了解海洋内部异常及其动力过程有重要意义。如何从现有的海洋表层遥感观测资料提取海洋内部关键动力环境信息场是具有挑战性的海洋遥感技术前沿。本文采用支持向量回归(SVR)方法,通过卫星遥感观测获取的多源海表参量(海表高度异常(SSHA)、海表温度异常(SSTA)、海表盐度异常(SSSA)和海表风场异常(SSWA)),选择最优参量输入组合,感知海洋次表层温度异常(STA),并用实测Argo数据作精度验证。结果表明SVR模型可准确估算全球尺度的STA(1000 m深度以浅);当SVR输入变量为2个(SSHA、SSTA)、3个(SSHA、SSTA、SSSA)、4个(SSHA、SSTA、SSSA、SSWA)时对应的平均均方差(MSE)分别为0.0090、0.0086、0.0087,平均决定系数(R2)分别为0.443、0.457、0.485。因此,除了SSHA和SSTA外,SSSA与SSWA的输入对SVR模型的估算有积极影响,有助于提高STA的估算精度。在全球增暖与减缓背景下,该研究可为从表层卫星遥感观测提取海洋内部热力异常信息研究提供重要技术支持,有利于拓展卫星对海观测范围。
Subsurface thermal structure of the global ocean is a key factor that reflects the impact of global climate variability and change. Accurately determining and describing the global subsurface and deeper ocean thermal structure from satellite measurements are becoming even more important for understanding the ocean interior anomaly and dynamic processes during recent global warming and hiatus. The extent to which such surface remote sensing observations can be used to develop information about the global ocean interior is essential but challenging.
This work proposes a Support Vector Regression (SVR) method, a popular machine learning method for data regression used to estimate Subsurface Temperature Anomaly (STA) in the global ocean. The SVR model can well estimate the global STA upper 1000 m through a suite of satellite remote sensing observations of sea surface parameters [including Sea Surface Height Anomaly (SSHA), Sea Surface Temperature Anomaly (SSTA), Sea Surface Salinity Anomaly (SSSA), and Sea Surface Wind Anomaly (SSWA)] with in situ Argo data for training and testing at different depth levels. In this study, we employed the Mean Squared Error (MSE) and squared correlation coefficient (R2) to assess the performance of SVR on STA estimation.
Results from the SVR model were validated to test the accuracy and reliability using the worldwide Argo STA data (upper 1000 m depth). The average MSE and R2 of the 15 levels are 0.0090/0.0086/0.0087 and 0.443/0.457/0.485 for two attributes (SSHA, SSTA)/three attributes (SSHA, SSTA, SSSA)/four attributes (SSHA, SSTA, SSSA, SSWA) SVR, respectively. The estimation accuracy was improved by including SSSA and SSWA for SVR input (MSE decreased by 0.4%/0.3% and R2 increased by 1.4%/4.2% on average). The estimation accuracy gradually decreased with the increase in depth from 500 m. With the increase in depth, the absolute value of STA became smaller, i.e., it became more indistinctive in the spatial heterogeneity. The STA became less intensive in the deeper ocean due to the water stratification and stability.
Results showed that SSSA and SSWA, in addition to SSTA and SSHA, are useful parameters that can help estimate the subsurface thermal structure and improve the STA estimation accuracy. Moreover, an obvious advantage for SVR is the absence of limitation on the input of sea surface parameters. Therefore, we can figure out more potential and useful sea surface parameters from satellite remote sensing as input attributes to further improve the STA sensing accuracy from SVR machine learning. This study provides a helpful technique for studying thermal variability in the ocean interior, which has played an important role in recent global warming and hiatus from satellite observations over global scale.