下载中心
优秀审稿专家
优秀论文
相关链接
摘要
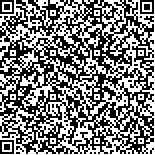
传统的实时异常探测算法需对高维的背景样本统计矩阵进行求逆运算,数值稳定性差、时间复杂度高。而基于Cholesky分解,将高维矩阵的求逆运算转换为求解下三角线性系统,采用Cholesky分解因子的一阶修正方法快速更新背景统计信息,降低逐像元实时处理的时间复杂度并且保持数值稳定性。由于算法仅涉及下三角矩阵的更新过程,压缩了数据存储空间,适用于机载或星上实时处理。采用3维接收器曲线(3D-ROC)以及计算机实际处理时间对实验结果进行定量化分析,结果表明,该算法在不降低异常探测精度的同时,对当前时刻像元的实时处理时间约缩短为基于QR分解算法的0.4%—0.65%,或减少至基于Woodbury矩阵引理算法的27%—33%,有效提高实时高光谱异常探测器的计算性能,并且保持处理过程中的数值稳定性。
关键词:
高光谱 异常探测 实时处理 Cholesky分解 一阶修正Anomaly detection is one of the most important issues in hyperspectral remote sensing. However, traditional anomaly detection algorithms cannot be used for onboard real-time processing due to heavy computational load caused by the dimensionality curse of hyperspectral data. To implement onboard real-time hyperspectral anomaly detection, the following must be performed: (1) conduct the process in a causal progressive manner without any future data relative to the pixel under test; (2) output the result for each sample right after collecting it; (3) process the data with constant theoretical computational complexity. The present widely used algorithms generally employ QR decomposition or Woodbury’s Identity for real-time anomaly detection. However, their computational load is still extremely high. Also, they suffered serious numerical instabilities led by runoff error in the matrix inverting process. The main objective of this study is to further accelerate the real-time detection process and avoid the matrix inversion module to maintain numerical stabilities.
In this work, we proposed a novel real-time sample-wise hyperspectral anomaly detection algorithm based on Cholesky decomposition. The background sample correlation matrix and covariance matrix were symmetric positive definite, which can be factored into a lower triangular matrix and its transpose. We modified the background suppression process into a process that finds the solution to a lower-triangular linear system based on this characteristic. Furthermore, the real-time process is significantly accelerated by virtue of rank-1 updates to the Cholesky factor of the background statistical matrix. Moreover, the numerical stabilities were maintained. Finally, we performed a three dimensional ROC (3D-ROC) analysis to evaluate the performance of real-time anomaly detection in terms of background suppression, detection power, false alarm, and the relationship between each other.
An experiment on an actual hyperspectral dataset collected by Field Imaging Spectrometer System (FISS) revealed the following. (1) The proposed algorithm significantly reduced the computing time to process the incoming data. The theoretical computational load demonstrated high efficiency of the technique developed in this work. The time consumption for each incoming sample was reduced to 0.4%—0.65% of the time consumed by traditional QR decomposition-based algorithms, and 27%—33% of the time corresponding to Woodbury’s identity-based techniques. (2) The sample varying background suppression provided an acceptable visual inspection of the anomalies. Real-time processing prevented these weak signals from being suppressed by later detected strong signals. Additionally, 3D-ROC analysis could effectively evaluate the detection performance of hyperspectral anomaly detectors.
The real-time sample-wise hyperspectral anomaly detector developed in this study is not only computationally efficient but also numerically stable, significantly contributing to onboard implementations.