下载中心
优秀审稿专家
优秀论文
相关链接
摘要
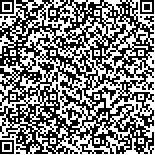
在样本数目稀少情况下实现高光谱图像精细分类是个挑战性的问题。高光谱图像信噪比提高比较困难,噪声大小对分类结果有最直接的影响。利用高光谱图像相邻波段之间的相关性和相邻像素之间的相关性,提出多级降噪滤波的高光谱图像分类方法,通过改进的两阶段稀疏与低秩矩阵分解方法,去除高光谱图像中能量较高的噪声,利用主成分分析方法去除高光谱图像中能量较低的噪声,引导滤波方法去除分类结果图中的“椒盐噪声”。选取两幅真实高光谱图像进行实验,结果表明,两阶段稀疏与低秩矩阵分解法和主成分分析法两种降噪方法具有较强的互补性;引导滤波方法使得分类图更加平滑且分类精度更高。与其他光谱空间分类方法相比,本文方法分类精度更高,且在样本极少时能获得很高的分类精度。
The fine-grained classification of hyperspectral image with small training samples is a major challenge for all kinds of classifiers. The signal-to-noise ratio of hyperspectral image is usually difficult to improve, and the magnitude of noise has a direct impact on classification results. Thus, noise reduction is one of the most important pretreatment measures for hyperspectral image classification. Employing the strong relevance between adjacent bands of hyperspectral images and the relevance between adjacent pixels in the space, a novel hyperspectral image classification method based on multi-level denoising and filtering is proposed.
One two-phase Sparse and Low Rank Matrix Decomposition (SLRMD) method is introduced to remove the noise with high energy. At the first phase, the hyperspectral image is segmented, and each patch will use the SLRMD method to perform noise reduction based on the spectral correlation between the pixels within the same patch. At the second phase, the pixels of all patches will be merged together for noise reduction based on the spectral correlation of the adjacent bands of the hyperspectral image. Secondly, then principal component analysis (PCA) is introduced to remove the noise with low energy. Thirdly, Support Vector Machine (SVM) is used to classify the de-noised and dimension reduced hyperspectral dataset. Finally, guided filter is introduced to remove the "salt and pepper noise" in the classification map.
We use the Indian Pines hyperspectral dataset as an example to verify the noise reduction effect of sparse and low rank matrix decomposition methods. The effect of image noise reduction is very obvious, and the bands after noise reduction show very strong correlation. The pixel spectrum of the original image contains a lot of noise information, especially in the first few bands and the last few bands, whereas the pixel spectrum of the low rank image becomes very smooth. The Spectral and Spatial De-Correlation (SSDC) and Local Variance Estimation (LVE) methods were used to evaluate the change of image quality before and after noise reduction. The signal-to-noise ratio of hyperspectral images is significantly improved after low rank matrix decomposition, especially at both ends of the spectral range. Two hyperspectral images, i.e., Indian Pines and University of Pavia, and some related classification methods are used for comparative experiments.The results show that the classification accuracy of our method is 25.85% and 13.2% higher than that of the SVM method, and 6.04% and 5.79% higher than the best method respectively.
The two-phase SLRMD method proposed in this paper has better strong noise removal effect than the conventional SLRMD method, and it is more helpful to improve the classification accuracy of hyperspectral image. Moreover, SLRMD, principal component analysis and guided filtering, these three noise reduction and dimension reduction methods are highly complementary, so they should be used together to improve the signal-to-noise ratio of hyperspectral image and make the classification results more natural and smooth.