下载中心
优秀审稿专家
优秀论文
相关链接
摘要
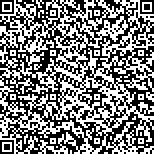
多尺度分割是遥感影像分析的关键步骤,影像分割过程中的尺度参数选择直接关系到面向对象影像分析的质量和精度。首先,总结了面向对象影像分析中尺度概念的内涵,分析遥感影像空间和属性两大基本特征,依据空间统计和光谱统计获得理论上最优的空间尺度分割参数、属性尺度分割参数。其次,运用了基于谱空间统计的高分辨率影像分割尺度估计方法,分析了分形网络演化多尺度分割与影像谱空间统计特征的关系,进而将基于谱空间统计的面向对象影像分析尺度参数应用于分形网络演化多尺度分割算法中,最后,对其参数的合理性进行验证。研究采用高空间分辨率IKONOS和SPOT 5影像数据,选择建筑实验区和农田实验区进行空间和光谱特征统计,以进一步估计分割中的最佳尺度参数。使用分形网络演化方法对图像进行分割,利用监督分类对本文提出的尺度估计方法进行验证,验证结果表明尺度估计方法可以一定程度上保证后续的面向对象影像分类的精度。不同于以往分割后评价的尺度选择方法会需要大量的运算量,本文方法不需要先验知识的参与,且在分割前就可以自适应地估计出相对较为合适的尺度参数,提高了面向对象信息提取的自动化程度。
Multi-scale segmentation is the key step of analysis in remotely-sensed imagery. Scale parameter selection in the segmentation process is directly related to the quality and accuracy of object-oriented analysis. Only on the basis of experience for segmentation parameter choice that has less quantitative analysis ways, currently. These methods lack quantitative estimation before segmentation, with considerable workloads and low efficiency. From the perspective of scientific research, the scientificity and universality of these methods are poor.
The objective of this paper is to use the quantitative method to determine the scale parameterin order to realize the automatic extracting target on the object-oriented analysis.
This paper summarizes the concept of scale parameter in the object-oriented analysis. The commonly used segmentation scale parameters into spatial and attribute bandwidths were also analyzed. This paper used a spatial and spectral statistics-based scale parameter selection method for object-based information extraction from high spatial resolution remote sensing images. The relationship between Fractal Net Evolution Approach (FNEA) in multi-scale segmentation and spatial statistical characteristics was analyzed. Scale estimation based on spatial and spectral statistical characteristics was applied to FNEA in multi-scale segmentation. Meanwhile, the scale estimation approach proposed in this paper was verified by high spatial resolution image, namely IKONOS and SPOT 5 data. Construction and farmland areas were selected for spatial and spectral statistical characteristics, respectively, to further estimate the optimal scale parameters in segmentation. A series of supervised classification was performed to verify the reasonability of the predicted optimal scale parameter.
The classification and accuracy assessment results show that the estimated scale by spatial statistical characteristics is basically close to the optimal one in the FNEA-based multi-scale segmentation. The proposed scale estimation approach can ensure the accuracy of the following object-oriented image classification.
The method can be used to estimate the appropriate scale parameters before segmentation. In addition, it is an essentially data-driven method that requires nearly no prior knowledge; thus, it can enhance the efficiency and automatic degree of object-based image analysis.