下载中心
优秀审稿专家
优秀论文
相关链接
摘要
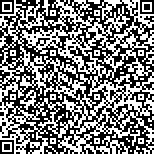
遥感图像数据的海量性、多样性和复杂性等特点对遥感图像检索的速度和精度提出了更高的要求,其中特征提取是影响遥感图像检索效果的关键。本文方法首先对遥感图像进行预处理,然后基于稀疏自动编码的方法在大量未标注的遥感图像上进行特征学习得到特征字典,基于卷积神经网络的思想,使用训练出来的特征字典对遥感图像进行卷积和池化得到每幅图像的特征图;接下来使用特征图训练Softmax分类器;最后对待检索图像分类,在同一类别中计算特征间的距离,进而实现遥感图像的检索。实验结果表明,该方法能够有效提高遥感图像检索的速度和准确度。
关键词:
遥感图像检索 深度学习 稀疏自动编码 卷积神经网络 Softmax分类器Massive data and diversity characteristics exert higher demands on the retrieval of remote sensing images. The feature extraction algorithm is the most outstanding factor that influences the performance of retrieval methods. Traditional feature extraction methods cause the problem of semantic gap, which occurs when the low-level feature does not perfectly reflect or match the purpose of retrieval. BOVW and multilayer cluster analysis have been proposed to solve semantic gap. However, the usability of these methods is limited given their dependence on classification or cluster algorithms and artificiality. A semi supervised deep-learning method was proposed in this paper. This method combines Sparse Auto encoder (SA) and the principle of Convolutional Neural Networks (CNNs).
The method involves four steps:first, the remote sensing images are pretreated with the ZCA whitening method. Second, the feature dictionary is extracted using SA, an algorithm that deals with nonannotated data. Subsequently, the feature dictionary is utilized in image convolution following the principle of CNNs, which imitates the neural net of organisms and decreases the number of features. Average pooling is conducted after image convolution. Both convolution and pooling are implemented to reduce model complexity, thus calculating distance faster. Third, the soft max classifier categorizes remote sensing images into five classes. Lastly, the remote sensing image retrieval is sorted based on the Euclidean distance between the query image and database in the same category as the query image.
Experimental results based on high-resolution remote sensing images demonstrate that the proposed method is effective and is more accurate than the methods that are based on color and texture features. Image classification before sorting speeds up retrieval by 27.6%. In addition, the semi-supervised deep learning algorithm is stable when the number of returned images increases. Given that the number of neurons in the hidden SA layer and the region size of pooling primarily affect retrieval results, this study conducted several optimization experiments on these two parameters. Moreover, the algorithm in this research performed well when the number of images in the data set and the retrieval accuracy on a larger data set increased, which are meaningful for the retrieval of massive remote sensing images.
The semisupervised deep learning algorithm decreases the time of image annotation, which is an exhausting job. Unlike traditional methods that extracts the features of color, texture, and shape, the method in this study directly extracts the feature dictionary from image elements with good accuracy. Moreover, the efficiency of the proposed method is guaranteed by convolution and pooling, which reduce the feature dimension. Furthermore, our experiment proves that this algorithm performs well in retrieving high-resolution remote sensing images.