下载中心
优秀审稿专家
优秀论文
相关链接
摘要
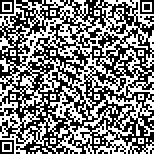
传统词包模型的视觉词典忽略了场景本身包含的类别信息,难以区分不同类别但外观相似的场景,针对这个问题,本文提出一种顾及场景类别信息的视觉单词优化方法,分别使用Boiman的分配策略和主成分分析对不同场景类别视觉单词的模糊性和单词冗余进行优化,增强视觉词典的辨识能力。本文算法通过计算不同视觉单词的影像频率,剔除视觉词典中影像频率较小的视觉单词,得到每种场景的类别视觉词典,计算类别直方图,将类别直方图和原始视觉直方图融合,得到不同类别场景的融合直方图,将其作为SVM分类器的输入向量进行训练和分类。选取遥感场景标准数据集,验证算法,实验结果表明:本算法能适应不同大小的视觉词典,在模型中增加场景类别信息,增强了词包模型的辨识能力,有效降低场景错分概率,总体分类精度高达89.5%,优于传统的基于金字塔匹配词包模型的遥感影像场景分类算法。
The traditional Bag Of Words (BOW) model disregards the scene label information of remote sensing images and ambiguity or redundancy of visual vocabularies. Hence, utilizing BOW to classify categories with similar backgrounds is unsuitable. Therefore, we propose an image scene classification algorithm based on the optimization of visual words with respect to scene label information to handle the said problem.
This paper reports on an image scene classification algorithm based on the optimization of visual words with respect to scene label information. The algorithm procedure is as follows:first, images are divided into patches utilizing Spatial Pyramid Matching, and then Scale Invariant Features Transform (SIFT) features are extracted for each local image patch. These features are then clustered with K-means to form a histogram of each patch at different levels utilizing the Boiman strategy. We adopt Image Frequency as the feature selection method on visual words in each category to eliminate visual vocabulary irrelevant to a specific category and obtain a class-specific codebook. Principal Component Analysis (PCA) is then utilized to eliminate redundant visual vocabulary. Finally, we produce a mixture of class-specific histograms in each image patch at different pyramid levels and a traditional histogram with an adaptive weight. A fusion of histograms will be placed in a Support Vector Machine (SVM).
We conducted experiments in this study on standard datasets of scene classification. Five experiments were conducted to demonstrate the performance of proposed algorithm. The first experiment shows that our algorithm performs better than methods that do not consider the scene label information with an increased accuracy of approximately 6 percent. The second experiment shows that the proposed method suitably performs in classifying categories with similar backgrounds and classifying error decreases in most categories. The third experiment demonstrates that the accuracy of the proposed method is higher at each pyramid level, and combined pyramids can offer even higher accuracy. The fourth experiment shows that method utilizing an adaptive weighted fusion method is more accurate than methods without. The final experiment demonstrates that the proposed algorithm performs better than other representative methods under the same conditions.
This study proposes a method based on the optimization of visual words with respect to scene label information. This algorithm extracts SIFT features at different levels of pyramids combined with the Boiman strategy to generate universal histograms. DF is adopted as the feature selection method to remove visual words irrelevant to a specific category. PCA is then applied to remove redundancy and obtain class-specific codebook and histograms. Finally, a practical adaptive weighted fusion method that combines the traditional histograms of different levels with the class-specific histogram is proposed and placed in an SVM trainer and classifier. The experiment results show that the proposed algorithm suitably performs in classifying categories with similar backgrounds and displays higher stability. However, the proposed algorithm only considers one SIFT descriptor that corresponds to only one visual word. We can perform experiments on one SIFT descriptor that corresponds to several visual words and other feature selection procedures in future research.