下载中心
优秀审稿专家
优秀论文
相关链接
摘要
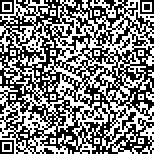
为提高高分辨率光学遥感图像港口自动检测的准确性,常需综合多类线索并进行复杂的特征提取、融合与分类推理,从而带来较高的计算复杂度。为此,仿生人类视觉注意机制,提出了一种复合线索视觉注意模型,综合利用高分辨率光学遥感图像港口多尺度底层特征和高层知识线索,实现了港口检测特征自然融合与综合分类推理。该方法在提高检测效果的同时较好地控制了计算量的增长,避免了复杂特征的大范围区域提取,采用多步快速算法降低了整个算法的计算复杂度,实现了计算资源受限条件下港口的快速定位与检测。同时,由于能将有限计算资源快速聚焦于最可能含有港口目标的区域,大大提高了目标检测方法响应的实时性。来自不同卫星的高分辨率光学遥感图像实验结果,验证了提出方法的有效性。
Harbor detection in high-resolution remote sensing images is a complex scene object detection problem, which is significant for applications such as harbor monitoring and space reconnaissance. This process is commonly requires complex feature extraction and analysis with high computational complexity. Existing works often select a smaller feature extraction region utilizing a scenario set according to experience and intuition and deal with a trade-off between computational complexity and object detection accuracy. The resolution and extraction order of features must be considered systematically to resolve this dilemma.
This paper reports on a complex cue visual attention model, which is a bionic human visual attention mechanism, for harbor detection in high-resolution remote sensing images. It combines multi-scale, low-level features with high-level knowledge clues in proper resolution layers of the Gauss pyramid of the input image. This model also performs natural feature integration and comprehensive classification reasoning for harbor detection. Multi-step fast algorithms are employed to lower the computational complexity of the entire algorithm.
Experiments in high-resolution optical remote sensing images from different satellites validate the proposed method. Non-harbor regions are immediately excluded from complicated feature extraction, whereas regions that most likely contain harbors are focused on for feature extraction and analysis. Thus, harbor regions are rapidly located and detected under the condition of limited computational resources. Detection effects are improved with complex feature extraction and analysis with a minimal increase in computational complexity.
The complex cue visual attention model proposed in this study considers the resolution and extraction order of features systematically. It lowers the computational complexity of traditional object detection methods largely without decreasing the detection accuracy and can be applied in other complex scene object detection problems.