下载中心
优秀审稿专家
优秀论文
相关链接
摘要
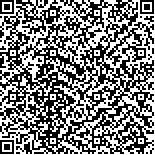
由于城市地表组成的复杂性,基于单核函数的支持向量回归模型很难满足精度。本文结合空间-光谱组合核函数和支持向量回归,提出了一种提取高光谱影像不透水面丰度的改进算法。首先从高光谱遥感图像上提取波谱特征和多通道灰度共生矩阵空间纹理特征,选取研究区10%像元特征数据作为训练数据,以线性加权求和核为多核组合方式,建立结合光谱信息和空间信息的组合核支持向量回归模型。然后,用生成的回归模型预测未知像元不透水面丰度值。最后,对实验结果进行评价。在模拟数据试验中,本文算法比单核回归均方根误差平均降低1.4%,决定系数比单核回归平均提高0.6%。在Hyperion数据两组试验中,该算法比单核回归均方根误差平均降低1.8%,决定系数比单核回归平均提高11.7%。模拟和真实两种高光谱数据实验中,本文算法均得到了空间形态上更准确的不透水面结果,单核回归结果存在失真现象。研究结果表明:本文算法能够有效提取城市不透水面丰度,与单核方法相比有较明显的精度提升。
Impervious surfaces, such as houses, cement or asphalt roads, parking lots, and other artificial surfaces, can be used as indicators for environment monitoring. Several methods have been proposed to estimate impervious surfaces by using remote sensing images. However, accurate extraction of impervious surfaces remains challenging because of the diversity of urban land covers. Thus, this paper presents an improved hyperspectral remote sensing algorithm for impervious surface extraction by combining spatial-spectral kernel and Support Vector Regression (SVR).
The composite kernel support vector regression model estimates impervious surface abundance by fitting an optimal approximating hyper plane to a set of training samples. Basing on the hyperspectral image, we first extract spatial-spectral feature and then select 10% pixels as training data. Spectral features include reflectivity of each pixels, NDVI, greenness and brightness of tasseled cap transformation, soil-adjusted vegetation index, and normalized difference built-up index. Gray level co-occurrence matrix approach is employed to extract spatial features. The first and second moments are identified as effective texture measures. The window size is set as 3×3 pixels for hyperspectral image. SVR method generally uses a single kernel. In this study, instead of using only one single kernel, spatial and spectral kernels are integrated into a kernel framework. Basic kernels include Gaussian, poly, and linear kernels. Using a linear weighted summation kernel as composite kernel combination method, we set the composite kernel SVR model in a manner that combines spatial and spectral features. The values of unknown pixels are predicted using the composite kernel SVR model. Finally, we evaluate the results of the experiments. Two accuracy indices, namely, root mean square error and coefficient of determination, are employed to assess the accuracy of impervious surface extraction.
To test the performance of the composite kernel support vector regression model, we conducted experiments on simulated and real hyperspectral datasets. We also compared the performance of the proposed composite kernel SVR with single kernel SVR. On the experiment of simulation range, the root mean square error of the proposed algorithm is lower whereas than the determination coefficient is higher than those of single kernel method (1.4% and 0.6%, respectively). On the Hyperion data experiment, the root mean square error of the algorithm is lower but the determination coefficient is higher than those of single kernel method (1.8% and 11.7%, respectively. On the two kinds of hyperspectral data experiments, the proposed algorithm can obtain spatially explicit results. Furthermore, a distortion phenomenon is observed in the results of single-kernel SVR algorithm.
We propose a composite kernel support vector regression model for impervious surface extraction using a hyperspectral image. The results indicate that our algorithm can effectively extract urban impervious surface and exhibits higher accuracy than the single-kernel SVR model. In our future work, we will focus on multisource remote sensing fusion through multiple kernels for impervious surface extraction.