下载中心
优秀审稿专家
优秀论文
相关链接
摘要
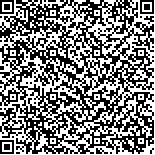
随机子空间集成是很有前景的高光谱图像分类技术,子空间的多样性和单个子空间的性能与集成后的分类精度密切相关。传统方法在增强单个子空间性能的同时,往往会获得大量最优但相似的子空间,因而减小它们之间的多样性,限制集成系统的分类精度。为此,提出优化子空间SVM集成的高光谱图像分类方法。该方法采用支持向量机(SVM)作为基分类器,并通过SVM之间的模式差别对随机子空间进行k-means聚类,最后选择每类中J-M距离最大的子空间进行集成,从而实现高光谱图像分类。实验结果显示,优化子空间SVM集成的高光谱图像分类方法能够有效解决小样本情况下的Hughes效应问题;总体精度达到75%-80%,Kappa系数达到0.61-0.74;比随机子空间集成方法和随机森林方法分类精度更高、更稳定,适合高光谱图像分类。
Promoting the accuracy of hyperspectral image classification is a crucial and complex issue. Hyperspectral image provides details of spectral variation of land surface with continuous spectral data. On the one hand, this characteristic is widely utilized to analyze and interpret different land-cover classes. On the other hand, the availability of large amounts of spectral space introduces challenging methodological issues, such as curse of dimensionality. Subspace ensemble systems, such as random subspace method (RSM), significantly outperform single classifiers in classifications involving hyperspectral image. However, two issues should be addressed to improve robustness and overall accuracy of the system. The first issue is diversity within subspace ensemble systems, and the second one is the classification accuracy of individual subspaces.
In this paper, we adopt Support Vector Machine (SVM) as base classifier and proposed a novel subspace ensemble method, namely, optimal subspace SVM Ensemble, for hyperspectral image classification to improve the performance of RSM. Based on random subspace selection as the initial step, a two-step procedure is designed to avoid similarity within ensemble systems during the optimization of individual subspace accuracy. Instead of maximizing the diversity of ensemble by using a specific diversity measure, the first step employs the k-means cluster procedure according to the similarity of SVM patterns to classify random base classifiers. Second, an optimization process is implemented with Jeffries-Matusita (J-M) distance as criterion by selecting the optimal subspace from each group in the formal phase. The final label is decided based on majority voting of optimal subspaces.
Experiments on two hyperspectral datasets reveal that the proposed OSSE obtains sound, robust, and overall accuracy compared with RSM and random forest method. In the first hyperspectral image, namely, the Pavia university data set, the maximum increases in Kappa coefficient and overall accuracy are about 0.04 and 2.64%, respectively, compared with those in RSM and about 0.15 and 12.75%, respectively, compared with those in random forest method. In the second hyperspectral image, namely, the Indian Pines data set, the maximum increases in Kappa coefficient and overall accuracy are about 0.02 and 1.00%, respectively, compared with those in RSM and about 0.13 and 11.12%, respectively, compared with those in random forest method.
The combination of optimal subspaces improves the diversity of subspace system and the accuracy of individual classifiers and thus exhibits better performance, particularly when using limited samples, which is common in hyperspectral image classification. Basing on the results of different parameter settings in OSSE, we found two interesting issues related to the number of clustering and initial size of random subspaces. First, the optimal number of clusters in OSSE is stable when using specific hyperspectral remote sensing data. Hence, the optimal number of cluster could be assessed using the characteristics of remote sensing images. Second, similar to RSM, increasing the number of random subspaces minimally contributes to the improvement of classification accuracy in OSSE. Consequently, to decrease the time cost of computing, we should avoid selecting numerous random subspaces.