下载中心
优秀审稿专家
优秀论文
相关链接
摘要
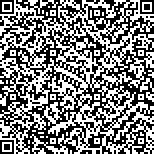
本文提出了一种新的混合像元聚集指数计算方法.该方法基于高分辨率遥感数据,采用方向孔隙率公式和线性混合模型相结合的方法,详细描述了混合像元内部由于植被覆盖度(FVC)、端元聚集指数以及叶倾角分布等引起的尺度差异.利用模拟数据进行初步敏感性分析,将计算得到的混合像元聚集指数与所占面积比例最大的端元聚集指数进行比较,结果表明端元聚集指数的不均一性对计算结果影响最大,由此得到的混合像元聚集指数较最大面积端元聚集指数下降了55%;植被覆盖度的不均一性影响次之,可使聚集指数降低43%;植被叶倾角分布(G函数)的空间异质性影响最小,约12%.敏感性分析的结果同时也证明了对于空间异质性较强的混合像元进行尺度差异修正的必要性.利用本文中提出的混合像元聚集指数方法有望在提高低分辨率叶面积指数反演中发挥重要作用.
A new algorithm for mixed-pixel clumping index (MPCI) calculation is proposed, which is based on the directional gap fraction and linear mixture model from the high-resolution remote sensing data. In this algorithm, the scale difference caused by the spatial heterogeneity of the fraction of vegetation cover (FVC), end-member clumping index (EMCI) and leaf inclination angle distribution (LAD) within the mixed pixel is well considered. The preliminary sensitivity analysis with the simulated data shows that the impact factors in a descending order are the special heterogeneity of EMCI, FVC and LAD (expressed as G function). The maximum values of the relative variation ratio caused by the heterogeneities are about 55%, 43% and 12%, correspondingly. The results also demonstrate that it is very necessary and signif icant to correct the scale difference in the mixed pixel with high special heterogeneity. It is potential to improve the accuracy of coarse leaf area index retrieval by employing the MPCI algorithm presented in this paper.