下载中心
优秀审稿专家
优秀论文
相关链接
摘要
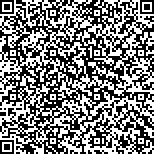
利用机载激光雷达点云数据,结合大量实测单木结构信息,分别从样地和单木尺度估算了森林地上生物量 AGB.首先,利用局部最大值单木提取算法提取了每个样地内的单木结构参数,并针对样地和单木尺度分别计算了一组激光雷达变量.然后,利用激光雷达变量和地上生物量及其两者的对数形式,从样地和单木尺度分别构建了估算模型.最后,针对两种尺度估算过程中存在的不确定性进行了详细讨论.结果表明:(1)样地和单木尺度模型估算的森林地上生物量与地面实测值都具有明显的相关性,且对数模型估算效果要优于非对数模型;(2)样地尺度模型估算效果(R2=0.84,rRMSE=0.23)明显优于单木尺度模型(R2=0.61,rRMSE=0.46);(3)按树木类型分别进行估算可以提高单木地上生物量的估算精度;(4)不论是样地还是单木尺度地上生物量估算都存在一定的不确定性,与样地尺度相比,单木尺度估算过程的不确定性更大,这种不确定性主要来自单木识别过程.
Forest Above-Ground Biomass (AGB) is a critical indicator of carbon cycle in the ecosystem. In this study, we estimated AGB at tree and plot levels using airborne LiDAR point clouds, together with numerous field-measured structure information of tree. We compared methodologies and analyzed uncertainties in the estimation of AGB at different spatial levels, to provide supportive suggestions for the management and monitoring of forest resources. First, LiDAR metrics were calculated at plot and tree levels. Tree-level LiDAR metrics were calculated on the basis of the results from individual tree delineation using local maxima algorithm. Second, stepwise regression models were established between LiDAR metrics and AGB and their logarithm-transformed data. Apart from regression models established by combining different tree species, regression models were also developed for two dominant tree species in our study area. Finally, uncertainty analyses were conducted on AGB estimation at plot and tree levels. LiDAR points with different point densities were generated using thinning strategy to analyze the effects of point density variation on Canopy Height Model (CHM), the basic data source for individual tree delineation. The number of plots with specific point density was counted. Results were as follows. (1) LiDAR-estimated AGB was highly correlated with the field-estimated ones at plot and individual tree levels. The logarithm-transformed models obtained higher estimation accuracy than the non-logarithm-transformed models. (2) Regression model developed at the plot level (R2=0.84, rRMSE=0.23) showed better performance than that at the tree level (R2=0.61, rRMSE=0.46). (3) Regression models built for the two dominant tree species improved the estimation accuracy of individual tree AGB; they obtained higher R2 (0.67 and 0.69) and lower rRMSE (0.29 and 0.21) than the combined models. (4) AGB estimation at plot and tree levels suffered from uncertainty problems. Not all the plots used in this study could obtain the same point density. CHM was seriously influenced by point density when the density was lower than seven points per square meter (pts/m2). The influence from point density decreased when the density reached 9 pts/m2. The influence can be simply identified from the visual appearance of CHMs and directly affected the calculation of LiDAR metrics and results of individual tree delineation. The conclusions were as follows. (1) Logarithm-transformed model can improve the estimation accuracy of AGB at plot and tree levels. (2) The estimation accuracy of tree AGB can be improved when regression models are established for different tree species. (3) Larger uncertainties exist in AGB estimation at the tree level compared with those at the plot level. These uncertainties mainly come from the process of individual tree delineation.