下载中心
优秀审稿专家
优秀论文
相关链接
摘要
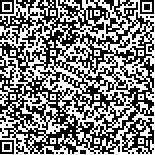
利用平均法、生物光学模型法和最优插值法3种数据融合方法对卫星传感器MODIS-Aqua、MOIDS-Terra及MERIS获取的南海叶绿素a浓度的数据进行了融合,通过比较融合产品的质量,对3融合方法进行了评价.(1)利用现场测量的南海遥感反射率和叶绿素a浓度数据建立了南海叶绿素a浓度的反演模型,并应用于MODIS-Aqua、MOIDS-Terra及MERIS Level 2反射率数据,获取南海叶绿素a浓度.(2)将平均法、生物光学模型法、最优插值法分别应用于上述3颗卫星的叶绿素a数据进行数据融合,并用现场测量的同步叶绿素数据对融合后的产品进行了印证.(3)利用3种融合方法分别对南海2011年MODIS-Aqua、MODIS-Terra和MERIS等3个传感器的叶绿素a数据进行了月融合,分析了融合的南海叶绿素a浓度的时空分布特征.结果表明,融合数据大幅度提高了空间覆盖率,且具有较高可信度.平均法、生物光学法和优化插等3种融合方法性能有较大的不同,生物光学法具有高的运行速度,但有时空间覆盖率仍不能满足要求;优化插值法具有高的空间覆盖率,但其运行速度较慢.因此,在具体应用中,应根据需求选择合适的融合方法.
The accurate analysis of temporal and spatial variation characteristics is significant for understanding the marine ecological system. Owing to the comprehensive effects of physical environment and biogeochemical effects in the South China Sea, chlorophyll distribution is characterized by a complicated, multi-temporal, and spatial scale. The South China Sea is often covered by clouds; especially in summer and autumn, cloud coverage is up to 80%. This characteristic causes the low coverage of optical sensor data in the South China Sea. Single optical sensor data cannot meet the demand of the study on the temporal and spatial characteristics of chlorophyll. The present study evaluates the performance of different merging methods with the chlorophyll data products of MODIS-aqua, MODIS-terra, and MERIS in the South China Sea. Long-term, continuous, and high-quality chlorophyll-a data are also provided for the study on the changes in ecological environment and biogeochemical cycle.
Three methods of ocean color data merging were used on the data products of MODIS-aqua, MODIS-terra, and MERIS to obtain the chlorophyll distribution in the South China Sea. The performance of the three merging methods was evaluated with in situ match-up data. An empirical inversion algorithm of the chlorophyll concentration was developed with the in situ measurements in the South China Sea. The algorithm was applied to retrieve the chlorophyll concentration from MODIS-aqua, MODIS-terra, and MERIS data. The three merging methods of averaging, bio-optical model, and optimal interpolation were used on the chlorophyll data from the three ocean color sensors in the South China Sea. The merging results were assessed with the in situ measurements and the previously known knowledge.The accurate analysis of temporal and spatial variation characteristics is significant for understanding the marine ecological system. Owing to the comprehensive effects of physical environment and biogeochemical effects in the South China Sea, chlorophyll distribution is characterized by a complicated, multi-temporal, and spatial scale. The South China Sea is often covered by clouds; especially in summer and autumn, cloud coverage is up to 80%. This characteristic causes the low coverage of optical sensor data in the South China Sea. Single optical sensor data cannot meet the demand of the study on the temporal and spatial characteristics of chlorophyll. The present study evaluates the performance of different merging methods with the chlorophyll data products of MODIS-aqua, MODIS-terra, and MERIS in the South China Sea. Long-term, continuous, and high-quality chlorophyll-a data are also provided for the study on the changes in ecological environment and biogeochemical cycle.Three methods of ocean color data merging were used on the data products of MODIS-aqua, MODIS-terra, and MERIS to obtain the chlorophyll distribution in the South China Sea. The performance of the three merging methods was evaluated with in situ match-up data. An empirical inversion algorithm of the chlorophyll concentration was developed with the in situ measurements in the South China Sea. The algorithm was applied to retrieve the chlorophyll concentration from MODIS-aqua, MODIS-terra, and MERIS data. The three merging methods of averaging, bio-optical model, and optimal interpolation were used on the chlorophyll data from the three ocean color sensors in the South China Sea. The merging results were assessed with the in situ measurements and the previously known knowledge.Merging products from the three merging methods have good consistency with the in situ match-up data. The accuracies of the merging products from the three methods are obviously different. Coverage of the merging data is significantly improved for all the three methods. Coverage of the data from averaging method and bio-optical model is similar, and the average of optimal interpolation is up to 100%. The running time of the three methods present a significant difference; the running times of averaging method and bio-optical model are similar, and are both approximately 40 times faster than optimal interpolation. Distributions of monthly chlorophyll products merged with MODIS-aqua, MODIS-terra, and MERIS from the three merging methods are in good agreement with previous studies.Coverage of the merged data is greatly increased, and the merged data have high reliability. The three merging methods have different performances. Bio-optical model has high running speed, while optimal interpolation has high coverage but low running speed. Averaging method and bio-optical model can keep considerable detailed information. In practice, the selection of merging method should depend on actual applications.
Merging products from the three merging methods have good consistency with the in situ match-up data. The accuracies of the merging products from the three methods are obviously different.
Coverage of the merging data is significantly improved for all the three methods. Coverage of the data from averaging method and bio-optical model is similar, and the average of optimal interpolation is up to 100%. The running time of the three methods present a significant difference; the running times of averaging method and bio-optical model are similar, and are both approximately 40 times faster than optimal interpolation. Distributions of monthly chlorophyll products merged with MODIS-aqua, MODIS-terra, and MERIS from the three merging methods are in good agreement with previous studies.Coverage of the merged data is greatly increased, and the merged data have high reliability. The three merging methods have different performances. Bio-optical model has high running speed, while optimal interpolation has high coverage but low running speed. Averaging method and bio-optical model can keep considerable detailed information. In practice, the selection of merging method should depend on actual applications.