下载中心
优秀审稿专家
优秀论文
相关链接
首页 > , Vol. , Issue () : -
摘要
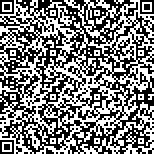
离子吸附型稀土矿因溶浸开采造成土壤污染,导致复垦植被长势差、成活率低,利用无人机影像监测有助于科学监管。然而矿区复杂环境下导致复垦植被整体特征差异较大使得无人机影像自动识别困难,识别准确率低。为了提高对无人机影像中矿区复垦植被单棵植株快速、精确的自动识别和定位,提出了融合全局特征YOLOv8n网络的矿区复垦植被检测方法(YOLOv8-AS)。该方法在YOLOv8n的基础上做了以下改进:(1)使用降采样模块ADown进行特征卷积操作,降低标准卷积随模型训练深度加深过程中导致的特征损失;(2)采用SPPF-GFP(Spatial Pyramid Pooling Fast-Global Feature Pool)模块进行特征提取,提高模型对整体特征差异较大的复垦植被的检测能力。结果表明:在自建复垦植被数据集上,YOLOv8-AS相较于YOLOv8n的mAP@0.5和mAP@0.5-0.95分别提升1.6%和2.4%;YOLOv8-AS的模型大小、参数量和浮点计算量较YOLOv8n分别下降了11%、10%和9%。YOLOv8-AS算法的mAP@0.5和mAP@0.5-0.95分别达到了91.1%、46.8%,相较于SSD、Faster R-CNN、RT-DETR、YOLOv5、YOLOv7和YOLOv7-tiny模型mAP@0.5分别提高了14.07%、23.32%、1.2%、2.3%、3.3%、2.9%和1.2%。此外,YOLOv8-AS能够针对小目标、简单和复杂场景进行快速、精准地检测复垦植被,同时较好地提升了对复垦植被单棵植株的识别和定位能力。该方法可为矿区生态恢复提供准确有效的技术支持。
The leaching mining of ion-adsorbed rare earth ore primarily employs in-situ leaching, pile leaching, and pool leaching methods, which result in significant soil pollution. This pollution presents serious environmental challenges, particularly affecting the growth and survival rates of reclaimed vegetation in rare earth mining areas. The restoration of reclaimed vegetation is crucial for mitigating environmental damage and restoring ecological balance. However, the application of intelligent technology to monitor and manage the health and growth of reclaimed vegetation in these mining areas encounters substantial challenges due to the complexities of the natural environment. Unmanned aerial vehicle (UAV) remote sensing image technology has emerged as a promising tool for monitoring and evaluating ecological restoration efforts in rare earth mining areas. The UAV can rapidly capture high-resolution images over large areas, facilitating efficient monitoring of reclaimed vegetation growth in these regions. However, the uneven spatial distribution, varying shapes and diverse overall characteristics of reclaimed vegetation present significant challenges for achieving high-precision automatic recognition from UVA images. Consequently, relying solely on traditional image processing technique for vegetation detection and classification proves to be be inadequate. To address these challenges and enhance the automatic recognition and localization capabilities of individual reclaimed vegetation in UAV images, this paper proposes a method for reclaimed vegetation detection in rare earth mining areas (YOLOv8n), which integrates the global feature YOLOv8-AS. This method represents an innovative improvement over YOLOv8n: first, the downsampling module ADown is introduced to optimize the feature convolution operation, thereby reducing the feature loss during the deep model training process. Second, the SPPF-GFP (Spatial Pyramid Pooling Fast - Global Feature Pool) module is employed for feature extraction, significantly enhancing the detection capability of reclaimed vegetation with substantial variations in overall features. The results showed that in the self-constructed rare earth mining reclamation vegetation dataset, YOLOv8-AS outperforms YOLOv8n by 1.6% and 2.4% in terms of mAP@0.5 and mAP@0.5-0.95, respectively. Compared to YOLOv8n, the model size, number of parameters, and floating point computation of YOLOv8-AS decreased by 11%, 10%, and 9%, respectively. The mAP@0.5 and mAP@0.5-0.95 for the YOLOv8-AS algorithm are 91.1% and 46.8%, respectively. When compared to SSD, Faster R-CNN, RT-DETR, YOLOv5, YOLOv7 and YOLOv7-TINY models regarding mAP@0.5, YOLOv8-AS shows improvements of 14.07%, 23.32%, 1.2%, 2.3%, 3.3%, 2.9% and 1.2%, respectively. According to the comparative experimental results of YOLOV8-AS and YOLOv8 across three scenarios—characterized by a predominance of small targets, simplicity, and complexity—the mAP@0.5-0.95 of YOLOV8-AS increased by 2.3%, 1.2%, and 3%, respectively, when compared to the baseline model YOLOv8. Furthermore, we applied YOLOv8-AS to the reclamation vegetation detection task in a larger scene within a rare earth mining area. The visualization results indicate that, regardless of the scenario—whether featuring numerous small targets, simple scenes, or complex environments—this method significantly enhanced its capacity to identify and accurately locate individual plants in the reclamation vegetation. This finding further substantiates its efficacy in accurately detecting reclaimed vegetation across various conditions. Such advancements are crucial for effectively monitoring the progress of ecological restoration in mining areas and provide essential support for achieving sustainable mining development.