下载中心
优秀审稿专家
优秀论文
相关链接
首页 > , Vol. , Issue () : -
摘要
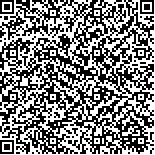
光学和合成孔径雷达(Synthetic Aperture Radar,SAR)图像由于成像原理的根本差异,影像间呈现出显著的辐射和几何差异,其自动精确匹配一直是当前国际学术研究的热点。目前,大多数基于深度学习的匹配方法聚焦于图像深度特征提取,但这些模型通常忽视了图像多尺度特征融合和共性特征表达,导致鲁棒性不足,难以应对复杂多变的地物场景。鉴于此,本研究提出了一种基于深度特征重构增强的光学和SAR图像鲁棒匹配方法。该方法构建了一个融合多尺度深度特征和图像特征重构的伪孪生共有特征提取网络。首先,通过多尺度特征提取架构,网络能够在像素级高效提取光学和SAR图像对的多尺度深度特征。其次,为光学图像设计了一个伪SAR图像翻译分支结构,利用深度特征重构图像,以增强网络学习更鲁棒的共性特征表达能力。最后,构造了基于多层特征匹配相似度和重构影像平均误差的联合损失函数,实现了光学和SAR图像的鲁棒匹配。在两种具有不同分辨率且包含多种地物场景(城市、郊区、荒漠、山地、水体)的遥感影像数据集上进行对比实验,结果表明,与当前几种最先进的匹配方法相比,所提出的方法均表现出最高的正确匹配率。
The automatic precise registration between optical and Synthetic Aperture Radar (SAR) imagery remains a significant challenge in remote sensing due to fundamental differences in their imaging mechanisms. The inherent modality gap manifests as substantial radiometric discrepancies (speckle noise vs. photometric consistency) and geometric distortions (side-looking geometry vs. nadir projection), posing critical obstacles for conventional feature matching approaches. While existing deep learning-based methods have made progress in extracting deep features, most architectures inadequately address two crucial aspects: multi-scale feature fusion across different imaging characteristics and cross-modal invariant feature representation, leading to compromised robustness in complex geographical scenarios. To address this, we propose a robust matching method based on deep feature reconstruction for optical and SAR images. Our method features a pseudo-Siamese network that integrates multi-scale deep features and image reconstruction. First, a multi-scale feature extraction architecture efficiently obtains multi-scale deep features at the pixel level. This architecture allows the network to capture detailed information from various scales, which is crucial for understanding the complex patterns present in remote sensing images. Second, a pseudo-SAR translation branch for optical images is designed to reconstruct images from deep features, enhancing the network"s ability to learn robust common features. This branch mimics the characteristics of SAR images, enabling the network to find shared features between the two image types more effectively. Through this translation process, the network learns to focus on the essential elements that are common to both optical and SAR images, thereby improving the matching accuracy. Finally, a joint loss function based on multi-layer feature matching similarity and reconstructed image average error is constructed for robust matching. This loss function ensures that the network not only matches features accurately across different layers but also maintains a high degree of fidelity in reconstructing the original images. By combining these two aspects, the network can achieve a balance between feature similarity and image reconstruction quality, leading to more reliable matching results. Experiments on two remote sensing image datasets with different resolutions and diverse terrain scenes (urban, suburban, desert, mountain, water) show that our method outperforms several state-of-the-art matching methods in correct matching rate. The proposed method demonstrates superior performance in various environments, indicating its versatility and effectiveness in real-world applications. The ability to handle different resolutions and terrain types is particularly important for practical remote sensing tasks, where conditions can vary widely. This research advances cross-modal image analysis by providing: 1) A new paradigm combining feature learning with cross-domain translation 2) Practical solutions for SAR-optical registration in challenging environments.