下载中心
优秀审稿专家
优秀论文
相关链接
首页 > , Vol. , Issue () : -
摘要
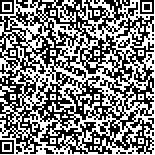
新疆维吾尔自治区拥有丰富的土地和光热资源,随着对可再生能源的需求增加及光伏技术的发展,新疆光伏电站装机容量位居全国前列。实时、准确识别光伏电站分布及其对周围植被空间聚集度的定量化结果可为新疆太阳能光伏选址提供数据及决策支持。本研究借助UNet、PSPNet(Pyramid Scene Parsing Network)、DeepLabV3+三种架构、八种骨干网络(ResNet-34、ResNet-50、ResNet-101、ResNet-152、MobileNetV2、DarkNet53、VGG16、Dense121)组合的深度学习语义分割模型,探究最优光伏电站识别模型,提取新疆地区光伏电站的空间分布。为研究光伏电站建设对植被空间聚集度的影响,计算了光伏电站周边从30 m至600 m等间隔分段的缓冲区内时间序列植被全局莫兰指数。结果表明:(1)基于UNet-ResNet50模型的光伏电站识别效果最优,准确率(Accuracy)为98.64%(>0.09%+)、F1分数(F1-score)为95%(>0.4%+)以及交并比(IOU)为90.47%(>0.57%+),优异的识别效果主要源于高质量的光伏样本集以及模型在特征提取和深度平衡方面的卓越表现。(2)利用Sentinel-2遥感影像和UNet-ResNet50模型提取了2020年新疆光伏电站,并将其划分为植被光伏和裸地光伏,面积占比分别为30%和70%。(3)距离光伏电站30 m至210 m不同缓冲区范围内,植被全局莫兰指数在2012到2020年间均呈显著下降趋势;距离光伏电站210 m至600 m缓冲区范围内,植被全局莫兰指数下降趋势明显减缓。距离光伏电站越近,植被空间聚集度受光伏电站影响越大,时间序列的下降趋势越明显。因此,开展光伏电站及其周围缓冲区的遥感监测,可为光伏电站的建设规划、精细化运营管理及其对生态环境的影响评估提供数据和技术支持。
The Xinjiang Uygur Autonomous Region, endowed with abundant land and solar energy resources, has emerged as a national leader in installed photovoltaic (PV) capacity, driven by the growing demand for renewable energy and advancements in PV technology. Accurate and real-time identification of PV station distribution, along with quantitative analysis of their effects on the spatial aggregation of surrounding vegetation, provides crucial data and informed decision-making support for PV siting in Xinjiang. This study utilizes deep learning semantic segmentation models that integrate three architectures—UNet, PSPNet (Pyramid Scene Parsing Network), and DeepLabV3+—with eight backbone networks (ResNet-34, ResNet-50, ResNet-101, ResNet-152, MobileNetV2, DarkNet53, VGG16, and DenseNet121). The objective is to determine the optimal model for photovoltaic (PV) station detection and to map the spatial distribution of PV stations across Xinjiang. To assess the impact of PV station construction on vegetation spatial aggregation, Global Moran"s I values were calculated as a time series within buffer zones divided into equal intervals, ranging from 30 m to 600 m around the PV stations. The results reveal that: (1) The UNet-ResNet50 model demonstrates superior performance in photovoltaic station recognition, achieving an accuracy of 98.64% (an improvement of 0.09 percentage points), an F1 score of 95% (an improvement of 0.4 percentage points), and an Intersection over Union (IoU) of 90.47% (an improvement of 0.57 percentage points). The exceptional recognition capabilities are primarily attributable to the high accuracy of the photovoltaic sample set and the model"s outstanding feature extraction and depth balancing abilities. (2) Utilizing Sentinel-2 remote sensing images and the UNet-ResNet50 model, the 2020 photovoltaic stations in Xinjiang were extracted and classified into vegetation-covered and bare land photovoltaic stations, with area proportions of 30% and 70%, respectively. (3) Within different buffer zones ranging from 30m to 210m from the photovoltaic station, the Global Moran"s I of vegetation shows a significant downward trend from 2012 to 2020. In the buffer zones 210m to 600m from the photovoltaic station, the downward trend of the Global Moran"s I of vegetation slows down significantly. The closer to the photovoltaic station, the greater the impact on the spatial aggregation of vegetation, and the more evident the downward trend in the time series. Therefore, remote sensing monitoring of photovoltaic (PV) stations and their surrounding buffer zones offers critical data and technical support for construction planning, precise operational management, and the assessment of ecological impacts associated with PV stations.