下载中心
优秀审稿专家
优秀论文
相关链接
首页 > , Vol. , Issue () : -
摘要
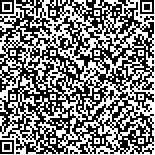
热带红树林是生产力最高、最富有生物多样性的森林资源之一,红树林森林资源调查能为森林资源的科学管理和保护提供数据支撑,准确的单木分割是二类森林资源调查的前提。地基激光雷达(Terrestrial Laser Scanning, TLS)能够提供海量、高精度的三维点云数据。然而,点云数据存在无规则、近密远疏且因遮挡导致的数据残缺等问题。并且,红树林场景复杂,冠层交错,树木互相遮挡,现有研究方法不适用于红树林TLS点云。本研究创新性地结合深度学习与传统算法,提出了一种针对红树林复杂场景TLS点云的高精度单木分割框架。该框架首先利用深度学习网络 RandLA-Net进行地面滤波和样地枝叶分离,其次通过连通域分割方法进行主干分割,最后通过提出的基于树顶点多约束模块实现单木分割。本文选取了中国海南七个红树林样地进行算法的精度评价,并与两种经典算法进行对比分析。实验结果显示,本文方法的总体精度达到0.87,大幅高于两种经典算法,证明了本文方法的有效性和可靠性。
“Objective” Tropical mangroves are one of the most productive and biodiverse forest resources but face several challenges such as monoculture planting structures, low ecosystem quality, low survival rates of planted mangroves, and threats from extreme weather and pests. Conducting surveys on mangrove forest resources provides essential data for the scientific management and conservation of these resources. Accurate segmentation of individual trees is a prerequisite for the inventory of such forest resources. Terrestrial laser scanning (TLS) can provide massive, high-precision, and high-resolution 3D point cloud data. However, the point cloud data are characterized by irregularities, varying densities due to distance, and incompleteness due to occlusions. Furthermore, the mangrove scene is complex with interlaced large and small trees and tree occlusions, making precise individual tree segmentation a considerable challenge. Traditional methods such as local maximum detection based on Canopy Height Models (CHM), have demonstrated good performance in simple plot scenarios. However, in the complex canopy interwoven environments of mangroves, where the upper canopy features are weak, these methods are less effective. Currently, there is a lack of research on individual tree segmentation algorithms for mangroves based on TLS point clouds. To address these issues, we aim to propose an individual tree segmentation algorithm applicable for complex mangrove scenes. “Method” This study innovatively combines deep learning and traditional algorithms to propose a high-precision individual tree segmentation framework for TLS point clouds in complex mangrove scenes. The framework initially employs the deep learning network RandLA-Net for ground filtering and wood-leaf separation. Subsequently, mangrove main stems are segmented using a connected component segmentation method. Finally, individual tree segmentation is achieved through the multiple tree tops constraint module. “Results” To assess the accuracy of the algorithm, we use three measures: completeness, correctness, and accuracy. We also conduct a comparative analysis with two classical algorithms. The experimental results demonstrate that the completeness of the proposed method across different mangrove plots is greater than 0.85, with an average of 0.90; the correctness of the proposed method is greater than the two classic algorithms in four plots; the mean accuracy of the proposed method in different sample plots reaches 0.87, which is significantly higher than the two classic algorithms, thus proving the effectiveness and reliability of our method. “Conclusion” This paper proposes an individual tree segmentation framework for TLS point clouds in complex mangrove scenes. Seven sample plots with various data characteristics were annotated to assess accuracy. The experimental results show that, compared to other algorithms, the proposed method achieved the highest accuracy. Despite the differing characteristics of the sample plots, the overall accuracy of the proposed method exceeded 0.8, demonstrating its effectiveness and robustness.