下载中心
优秀审稿专家
优秀论文
相关链接
首页 > , Vol. , Issue () : -
摘要
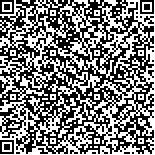
作者 | 单位 | 邮编 |
段德鑫 | 上海交通大学 电子信息与电气工程学院 | 200240 |
路遥 | 中国人民解放军61646部队 | |
黄立威 | 中国人民解放军61646部队 | |
刘佩林 | 上海交通大学 电子信息与电气工程学院 | |
文飞* | 上海交通大学 电子信息与电气工程学院 | 200240 |
与第二代人工神经网络(ANN)相比,第三代类脑脉冲神经网络(SNN)由于其高能效、高仿生、可解释等特点,在遥感影像智能处理的高能效、高精度、高可解译方面具有较大的潜在优势。针对现有脉冲神经网络算法延时较大的问题,本文提出一种基于类脑脉冲神经网络的遥感图像检测算法。该算法首先搭建了一个带有动态裁剪阈值激活函数的目标检测神经网络作为源网络进行预训练,随后借助训练过程中得到的裁剪阈值,通过激活神经元与脉冲神经元的映射关系将源网络转换为类脑脉冲神经网络,在继承源网络较高精度的同时还具备了低延迟、高仿生的特点。在SAR-Ship-Detection-Datasets(SSDD)和RSOD两个公开遥感数据集上的实验结果表明,该方法能够以极低的损失将源网络转换至类脑脉冲神经网络,并能在低时间步下对遥感目标实现较高的检测识别精度。同时该方法能够在继承ANN网络易于训练的特性与精度优势的同时,充分展现SNN的高稀疏度的带来的巨大能效优势。
(Objective) In the realm of intelligent processing of remote sensing images, the third-generation brain-inspired spiking neural network (SNN) surpasses its predecessor, the second-generation artificial neural network (ANN), with remarkable advantages in terms of high energy efficiency, high precision, and high interpretability. These advantages stem from the SNN"s characteristic features, including superior energy efficiency, elevated sparsity, and remarkable bio-plausibility. The integration of these features in the SNN presents an enthralling solution to the challenges faced in remote sensing image processing and holds immense potential for advancing this field further.(Method) The proposed algorithm introduces a novel approach by initially establishing a target detection neural network, which serves as the source artificial neural network for pre-training. This source network utilizes a dynamic clipping threshold activation function to optimize its performance. Subsequently, the algorithm transforms the source network into a brain-inspired spiking neural network by leveraging the mapping relationship between activated neurons and spiking neurons. This conversion process effectively incorporates the clipping thresholds obtained during training. By seamlessly transitioning the source network into an SNN, the algorithm ensures the preservation and enhancement of key characteristics essential for remote sensing image processing.(Result) To evaluate the efficacy of the proposed method, extensive experiments were conducted on two widely recognized open remote sensing datasets, namely SAR-Ship-Detection-Datasets (SSDD) and RSOD. The experimental results highlighted the exceptional capabilities of the proposed method in transforming the source network into a brain-inspired spiking neural network, with negligible loss in performance. Furthermore, the transformed SNN exhibited remarkable accuracy in detecting and recognizing remote sensing targets within significantly reduced time steps. The performance achieved by the transformed SNN was comparable to that of the source artificial neural network while tremendously reducing power consumption by over two magnitudes. This outcome highlights the immense potential of the proposed method in revolutionizing the field of remote sensing image processing by delivering high precision and interpretability, paired with significantly reduced energy consumption. (Conclusion) The integration of the third-generation brain-inspired spiking neural network (SNN) into the domain of remote sensing image processing holds tremendous potential, primarily due to its remarkable advantages in high precision and low energy consumption. The proposed algorithm highlights the distinctive attributes of the SNN, such as its low delay, high bionics, and the ability to inherit the high precision observed in the source network. These characteristics are promising indicators of the SNN"s capability to significantly enhance the intelligent processing of remote sensing images.By leveraging the SNN"s high precision and embracing bio-plausible principles, the proposed algorithm lays a robust foundation for future advancements in the field of remote sensing. The SNN"s inclusion in the image processing pipeline brings forth a paradigm shift, challenging traditional assumptions and unlocking new possibilities. The application of the SNN in remote sensing target detection offers high accuracy in identifying and classifying targets with remarkable precision. In conclusion, the proposed algorithm exhibits characteristics of low delay and high bionics while inheriting the high precision of the source network. This indicates its potential to significantly improve the intelligent processing of remote sensing images, offering high accuracy, precision, interpretability, low energy consumption and high bio-plausibility.