下载中心
优秀审稿专家
优秀论文
相关链接
首页 > , Vol. , Issue () : -
摘要
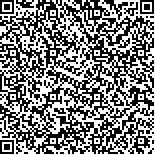
建筑物变化检测是遥感影像智能解译中的重要研究问题,针对跨域变化检测算法中存在的像素级对比学习噪声过大和目标域样本利用不充分等问题,本文提出了实例级对比学习域适应变化检测算法ICDA-CD。首先,使用区域级域混合替代实例级域混合,以实现源域和目标域图像的有效混合;然后,利用实例级对比学习,降低伪标签噪声的影响。具体来说,在编码器中,拉开变化实例区域双时相特征距离,并在解码器中,拉近各个变化实例特征之间的距离,这可以显著提升模型对源域和目标域特征表示的一致性;最后,在损失计算部分使用伪标签质量估计,使得低置信度区域的像素也可以参与训练,提高了目标域样本的利用率。实验表明,本文所提出来的方法在LEVIR-CD域迁移至S2Looking和S2Looking域迁移至LEVIR-CD时F1分数分别达到了43.91%和74.75%,优于几种先进的算法。
Building change detection is an important research problem in the intelligent interpretation of remote sensing images, and to address the problems of excessive pixel-level contrast learning noise and insufficient utilization of target domain samples in cross-domain change detection algorithms, this paper proposes the instance-level contrast learning domain adaptation change detection algorithm ICDA-CD. first, using region-level domain blending instead of instance-level domain blending to achieve effective blending of source and target domain images Then, using instance-level contrast learning to reduce the effect of pseudo-label noise. Specifically, in the encoder, pulling apart the bi-temporal feature distances in the change instance region and, in the decoder, pulling together the distances between individual change instance features can significantly improve the consistency of the model"s representations of source and target domains features; finally, using pseudo-label quality estimation in the loss calculation part allows pixels in low confidence regions to participate in the training, and the utilisation of the target domain samples is improved. Experiments show that the proposed method achieves F1 scores of 43.91% and 74.75% for transferring LEVIR-CD domain to S2Looking and S2Looking domain to LEVIR-CD respectively, outperforming several advanced algorithms.