下载中心
优秀审稿专家
优秀论文
相关链接
首页 > , Vol. , Issue () : -
摘要
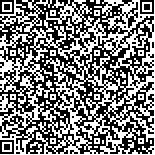
实景三维是重要的国家数字基础设施,其将地理信息从传统二维平面扩展到信息更丰富更全面的三维空间,数据以显式三维模型的形式存储表达,但经典的显式三维模型具有数据量大、可视化效果粗糙等问题,在一定程度上限制了其实际应用。神经辐射场(Neural Radiance Field,NeRF)是一种基于神经隐式立体表达(Neural Implicit Volume Representing)进行可微渲染(Differentiable Rendering)以实现高质量视图合成的新方法,被Mildenhall等(2020)首次提出,以其逼真的视图合成效果与新颖的实现方式成为计算机视觉领域的热点研究方向。自提出以来,国内外爆发式涌现出大量有关神经辐射场的研究文献,其中有少量研究开始探索将其用于大规模实景三维场景可视化,主要聚集于可视化效果的生成方法。本文回顾了神经辐射场提出的背景,介绍了神经辐射场及其在大规模实景三维可视化方面的研究进展,分析了目前利用神经辐射场进行大规模实景三维场景可视化研究中被关注的无边界场景、锯齿效果、瞬态遮挡、光度一致性、场景重照明与可见性场等问题,指出了目前研究在多源数据融合、视觉效果优化、虚拟环境感知等方面面临的挑战,对未来值得进一步探索的方向提出了见解。
Real-scene 3D is an important national digital infrastructure, which extends geographic information from 2D to 3D. The real-scene 3D data is stored and expressed in the form of explicit 3D model, which has the problems of large amount of data and rough visualization effect. Neural radiance field (NeRF), realizing differentiable rendering based on neural implicit volume representation, is a new approach of high-quality view synthesis. After being firstly proposed by Mildenhall et al. (2020), NeRF has become one of the hotest research directions in the field of computer vision due to its realistic view synthesis effect. A large number of literature about NeRF have been published since NeRF was proposed, and the application of NeRF in large-scale real-scene 3D visualization has also begun to attract the attention of some published papers. View synthesis is a novel way to realize the representation of 3D scenes, which uses sparse 2D images to generate realistic new views at any viewpoint in 3D space without the reconstruction of 3D models. The development of view synthesis technology has gone through several stages: image mosaicing, reprojection of 3D models, view interpolation, and volume representing technology. NeRF, as a new approach of view synthesis, samples 5D coordinates (location and viewing direction) along camera rays, feeds those locations into a multi-layer perceptron network to produce color and volume density, and use volume rendering techniques to composite these values into a new image. NeRF not only produces significantly higher quality rendering than prior volumetric approaches, but also requires just a fraction of the storage cost of other sampled volumetric representations. However, it faces the problems such as requirements for high quality of source data, failure to support for dynamic objects, low efficiency in processing, and single type of render target. Also, NeRF-related researches are mostly carried out based on laboratory environment or standardized data at present. Due to the drawbacks mentioned above, there exist many obstacles before applying NeRF to large-scale real-scene 3D visualization . This paper also reviews the workaround of unbounded scene, aliasing, luminosity consistency, scene re-lighting, and visibility field in the Block-NeRF algorithm, a variant of NeRF that can represent large-scale environments (Tancik et al. 2022). The Block-NeRF algorithm splits the environment into a set of Block-NeRFs that can be independently trained in parallel and composited during inference, and it selects relevant Block-NeRFs for rendering, which are then composited in a smooth manner when traversing the scene. To aid with this compositing, the Block-NeRF optimizes the appearances codes to match lighting conditions. It trains individual Block-NeRFs using techniques such as appearance embeddings, learned pose refinement, exposure input, transient objects, and visibility prediction. Nowadays, the studies of applying NeRF to large-scale real-scene 3D visualization are being carried out extensively, which has attracted a lot of attentions and plays a pioneering and leading role in further research. The results of these studies achieve the most basic 3D visualization effect of large-scale real-scene, but they are also limited by some conditions and their universality needs to be strongly improved. Since NeRF is still far from practical applications in producing large-scale real-scene 3D visualization, any little progressive exploration is likely to become a continuous research hotspot. As conclusion, this paper points out the challenges of NeRF research, including multi-source data fusion, visual effects optimization, virtual environment perception, which are waiting for more researchers.