下载中心
优秀审稿专家
优秀论文
相关链接
摘要
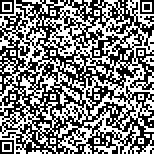
针对当前遥感图像舰船目标检测精度不佳问题,本文构建舰船目标数据集STAR,提出基于Dense RFB和LSTM多尺度舰船目标检测算法。该算法首先在SSD网络基础上设计了浅层特征增强模块,基于人眼视点图采用Dense RFB特征复用和膨胀卷积增大感受野的尺度和种类,增强浅层网络对细节特征的提取能力;其次设计了深层多尺度特征金字塔融合模块,采用FPN和LSTM思想,基于反卷积和残差网络对深层不同尺度特征进行融合,增强网络结构非线性和特征层的表征能力;最后加入聚焦分类损失函数进行联合训练,有效避免了正负样本失衡问题。在遥感图像舰船数据集上实验,本文所提舰船目标检测算法精度均值达到
Ship detection plays a crucial role in various applications and has drawn increasing attention in recent years. Deep learning methods based on CNNs, particularly SSD, have greatly improved detection performance due to their highly efficient feature extraction capability. However, SSD still has two problems. For instance, the detection network of arbitrarily arranged ship targets lacks a connection between high and low-level features and ignores contextual semantic information. Another problem is that natural factors such as light and clouds affect remote sensing images, thus ship detection may cause an imbalance of positive and negative samples.Aiming at solving the above issues, this paper proposes to achieve ship detection in remote sensing images by using a method based on Dense RFB and LSTM. This proposed method includes three elements. First, to enhance the detail feature extraction capability, this proposed method introduces a shallow feature enhancement module. This module draws on the idea of the human viewpoint, which uses Dense RFB feature reuse and expansion convolution to increase the receptive field. Second, to effectively extract deep semantic information and enhance the expressive ability of the network feature layer, a deep multi-scale feature pyramid fusion module (MFPF) is designed, as this proposed method draws on FPN and LSTM deconvolution and residual structure fuse deep multi-scale features. Finally, to solve the imbalance of positive and negative samples, the focal classification loss function is added, improving the accuracy of ship detection during training.The experiments were carried out on an optical remote sensing image dataset, in which only the ship dataset was used for training, validation, and testing. Results indicate that the proposed algorithm achieved an Average Precision (AP) of 81.98% and the detection speed reached 29.6 fps for ship targets, in which most ships were detected successfully. Moreover, for blurred, occluded, and partially-cropped ship targets, the algorithm’s detection effect is better than the traditional algorithm. Qualitative and quantitative results indicate that the generalization capability of the proposed method enhances ship detection.From this paper, we can draw three conclusions: (1) The proposed method can improve the extraction of detailed features and increase the receptive fields. (2) The focal loss function method shows good generalization capability. (3) The rotating box detection method is suitable for multi-scale and densely-arranged remote sensing images.