下载中心
优秀审稿专家
优秀论文
相关链接
摘要
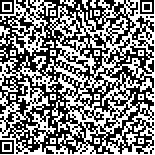
超分辨率重建是当前卫星遥感数据空间分辨率提升的重要技术,但目前现有的超分辨率重建方法在处理具有复杂地物特征的影像时效果往往不佳。当遥感影像中包含有各种非均匀地物信息时,难以构建一种通用的模型来解决遥感影像的病态问题。基于此,本文结合图像稀疏表达与非凸高阶全变分理论,提出了一种混合稀疏表示模型的新型超分辨率重建方法(MSR-SRR)。这种方法以遥感图像在多重变换域的稀疏性表达作为先验概率模型,通过正则化方法来完成超分辨率重构,不仅保留了超分重建结果影像的边缘信息,而且对影像中产生的“阶梯效应”进行了适当的平滑处理。该方法利用迭代重加权
When processing remote sensing images with complex features, the conventional Super-Resolution Reconstruction (SRR) methods are often not ideal, especially for remote sensing images containing various non-uniform object information. A universal method to solve this problem is difficult to construct at present. A new SR reconstruction method of mixed sparse representation model (MSR-SRR) combined with the sparse representation and non-convex high-order total variational regularizer has been proposed to solve this problem. In this method, the sparse representation of remote sensing images in multiple transform domains is regarded as a prior probability model, and the SR reconstruction is completed by regularization. The obtained image not only retains the edge information of the image result by SR reconstruction, but also smoothens the “ladder effect” of the image. The efficiency of operation and the quality of SR reconstruction results are improved by an effective re-weighted l1 alternating direction method. Results show that the sharpness of the image increases by 31.74% on the average, the half-peak width of PSFs is the largest, and the Gaussian variance value reaches 1.8415. The GF-4 satellite images have been selected to carry out validation experiment to verify the feasibility and validity of MSR-SRR. The reconstruction results show that the images using the MSR-SRR method have better definition, richer details, and higher quality than those with non-uniform interpolation, the POCS method, and IBP method. The support vector machine method is used to classify and evaluate the accuracy of the images before and after SR reconstruction. The results show that the overall accuracy and Kappa coefficient of the reconstructed super-resolution image are improved more significantly than the original image classification results. The OA value increases by 5.96%, and the Kappa coefficient increases by 9.7%. The findings confirmed that the MSR-SRR method is effective and feasible and has extensive practical value.