下载中心
优秀审稿专家
优秀论文
相关链接
摘要
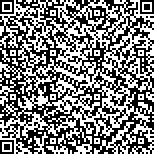
高光谱遥感影像数据具有高维特征、信息冗余、不确定性显著、小样本、空谱合一等特征,对其进行数据处理面临巨大挑战,高光谱遥感影像降维是高光谱遥感的重要研究方向之一。本文对当前高光谱遥感影像降维的相关研究进展进行了综述,在介绍高光谱遥感数据特点的基础上,重点从特征提取和特征选择两方面对高光谱遥感影像降维的最新研究和前沿进展进行了系统性综述;并从特征可分性、特征质量评价、特征数目确定、多特征优化以及需求驱动的特征选择等方面分析了高光谱遥感影像降维面临的挑战。随着智能化高光谱遥感的发展,高光谱遥感影像智能降维成为未来的发展方向,同时其发展将兼顾多特征质量评估与优选、搜索策略优化、满足应用需求等多目标的需求。随着高光谱遥感数据获取能力的提升和深入应用,高光谱遥感影像降维将会发挥重要而不可替代的作用。
Hyperspectral imaging can provide narrow bands and continuous spectrum information. However, hyperspectral image data have the characteristics of high dimensionality, rich features, information redundancy, small samples, and significant uncertainty, which result in difficulties in hyperspectral image data processing. Dimensionality reduction of hyperspectral remote sensing is one of the important topics in hyperspectral image data processing. Hyperspectral image data have hundreds of bands and can provide rich information, but a strong correlation exists between different bands, resulting in data redundancy. Therefore, the dimensionality problem is encountered during the processing of hyperspectral data, such as the increase in time complexity and the overfitting of the prediction model due to the increase in spectral feature dimension. More importantly, the number of training samples available for hyperspectral remote sensing images is small, and the feature dimension is much larger than the training sample. The classification accuracy will increase first and then decrease with the increase of feature dimensionality, that is, the “Hughes” phenomenon. Therefore, exploiting the rich information of hyperspectral images data and solving the problem of high feature dimension through certain methods have become key issues in the research on hyperspectral imaging data processing. The dimensionality reduction of hyperspectral remote sensing image is an approach to reduce the dimensionality of hyperspectral imaging through feature extraction or band selection while retaining as much effective information or features as possible. Feature extraction methods, such as principal component analysis, linear discriminant analysis, independent component analysis, manifold learning, and deep learning-based methods, use the projection transformation method to map hyperspectral data from high-dimensional space to low-dimensional space. Feature selection eliminates redundant bands without changing the original feature structure and finds representative feature band subsets, such as the selection based on information measurement and feature correlation. With the development of new technologies, evolutionary and intelligent algorithms, such as the genetic, ant colony, and firefly algorithms, have been applied in hyperspectral remote sensing dimensionality reduction.This article systematically summarizes and reviews the current advances in dimensionality reduction for hyperspectral remote sensing, especially for feature extraction and selection. For feature extraction, we review the advances of feature extraction algorithms based on index and parameters, projection and transformation, band combination, spatial algorithm, manifold learning, and deep learning. For band selection, the advances in information measurement, search strategy, optimized band number, multi-feature quality assessment, and optimization algorithms are reviewed. The challenges of dimensionality reduction for hyperspectral remote sensing are analyzed from five aspects: feature separability, feature quality evaluation, feature number determination, multi-feature optimization, and problem-oriented feature selection. Intelligent dimensionality reduction will be one of the most popular topics with the development of intelligent hyperspectral remote sensing. Meanwhile, multi-feature quality assessment, search strategy optimization and application requirements will attract special attention in the future. The dimensionality reduction of hyperspectral remote sensing will play an important and irreplaceable role in hyperspectral image data acquisition and applications.