下载中心
优秀审稿专家
优秀论文
相关链接
摘要
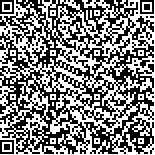
城市作为高密度建筑区域,在较小范围内有大量结构相似的建筑紧密分布。当前从高分辨率图像中准确检测建筑仍然是一个挑战,本文受边缘检测网络启发,提出一种强化边界精度的建筑物提取新方案,根据建筑物及边界特点改进深度网络,结合自下而上分组的分水岭分割提高分类精度和建筑边界的准确度。首先对数据预处理,生成建筑边界和建筑分割线两类辅助标签;改进性能较优的建筑检测框架ICT-Net网络,修改网络结构和损失函数,针对两类辅助标签,强化边界影响,提高网络性能;最后对网络预测结果应用结合分水岭分割和梯度提升回归树的后处理,实现高精度的建筑提取。结果表明,数据预处理、改进深度学习算法可提高建筑检测像素精度IOU(Intersection over Union)约1%。后处理能充分利用网络输出的概率信息,有效优化建筑边界,在网络预测结果的基础上提高建筑实例召回率10.5%。本文方案与原始的ICT-Net网络相比,提高建筑实例召回率22.9%。
High-density urban cities contain numerous similar buildings positioned in close proximity. Building detection from high-spatial-resolution remote sensing imagery in such scenes remains a challenge in computer vision and remote sensing urban applications. The integration of traditional segmentation algorithms and a novel neural network is an effective approach for such challenging settings. Inspired by the recent success of deep-learning-based edge detection, a new building detection method aiming at accurate boundaries is proposed. In accordance with the characteristics of buildings and their border, this study improves the network structure and integrates the network with bottom-up watershed segmentation to improve boundary precision and classification accuracy.First, two auxiliary labels, namely, the building boundary and parting line, are derived from the original dataset through data preprocessing. Second, the newly proposed building detection frame called ICT-Net is improved by modifying its structure and loss function in accordance with the two auxiliary labels to obtain the probability of three classes. Lastly, a post-process integrating watershed segmentation with gradient-boosted regression trees is employed to achieve high-accuracy building detection. Specifically, a probability feature map is generated by merging the probability of three classes. Watershed segmentation with building marker thresholds is applied to obtain building instances from the probability feature map. Then, the building probability of each building instance predicted by gradient-boosted regression trees is used to select building instances, resulting in building detection results. Parameter selection is also implemented.The performance of the proposed method is validated on the INRIA dataset, which provides aerial orthorectified color imagery with a spatial resolution of 0.3 m and with corresponding ground truth labels for two semantic classes: building and not building. Experimental results suggest that data preprocessing and the application of boundary loss can obtain an improvement of 1% in terms of the Intersection over Union (IOU) of building detection. The post-process can take full advantage of probability information from the network, thereby effectively optimizing the building boundary. The post-process brings an improvement of 10.5% in terms of building instance recall compared with the results of the neural network. Our study achieves a building instance recall rate that is 22.9% higher than that of the original ICT-Net.A novel building detection method based on a boundary-regulated network and watershed segmentation is proposed in this study. Experimental results reveal the advantages of the enhanced-boundary-oriented data preprocessing and modified neural network and demonstrate that the proposed method can further improve prediction accuracy on a network basis. However, the excellent performance of the proposed method largely depends on parameter selection, and further improvements should be made in the future.