下载中心
优秀审稿专家
优秀论文
相关链接
摘要
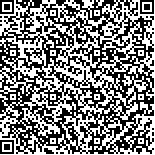
及时准确地获取耕地空间分布数据对于农业生产管理、产量估算、种植结构调整等具有重要意义。目前的耕地提取多基于多时相中低分辨率影像或单时相高分辨率影像,难以满足耕地破碎,农作物种植模式复杂的区域精度需求。基于此,本研究通过协同国产高分一号(GF-1)、高分二号(GF-2)和高分六号(GF-6)卫星影像,探索米级分辨率尺度下的耕地高精度提取方法。该方法以深度神经网络UNet为基础,通过协同GF-1/6的多时相优势和GF-2影像的高空间分辨率构建了CEUNet(Cropland Extraction UNet)模型,以充分挖掘耕地的时相特征和空间几何特征。同时,将基于CEUNet模型提取的米级耕地结果分别与基于UNet和多源不同分辨率遥感影像的语义分割(UNet_m)、基于UNet和单时相高分辨率影像的语义分割(UNet_s)、基于对象的随机森林分类(OBIA)、基于像元的随机森林分类(RF)提取的耕地结果展开对比,分析所提出的方法在不同区域的适宜性。结果表明,基于CEUNet模型提取的米级耕地总体精度达到92.92%,且基于CEUNet提取的耕地的逐像元验证结果在平均F1-Score值上相较于基于对象和基于像元的随机森林分类分别提升了0.21和0.21,相较于UNet_m和UNet_s分别提升了0.04和0.11,其中针对地块破碎,景观异质性高等区域,CEUNet相较于UNet_m和UNet_s提升了0.09和0.26。本研究提出的CEUNet模型能够充分发挥多源国产高分卫星数据的空间和时间优势,两者结合能够快速、高效地提取不同农业景观及不同种植模式的耕地空间分布信息。
Timely and accurate estimation of the spatial distribution of cropland is critical for agricultural production management, yield estimation, and planting structure adjustment. Previous studies on cropland mapping mostly focused on using moderate-/low-spatial-resolution images or single-phase high-spatial-resolution images, in which croplands in regions with fragmented landscapes and complex crop planting patterns are challenging to extract. The multi-source Gaofen (GF) satellites launched by China can provide images with high spatiotemporal resolution, thus presenting great potential for fine-scale cropland mapping with high accuracy. This study utilized GF-1, GF-2, and GF-6 satellites to explore a high-accuracy cropland mapping method at metric spatial resolution. Specifically, Cropland Extraction UNet (CEUNet) was developed based on the structure of UNet by integrating multi-temporal information from GF-1/6 and spatial details from GF-2 to fully exploit the spatial and temporal characteristics of cropland.To make full use of the details provided by high-spatial-resolution images, CEUNet adopted the same encoder structure as UNet. It consisted of the repeated application of two 3×3 convolutions (unpadded convolutions), each followed by a 2×2 max pooling operation with stride of 2 for down sampling. The input image size was gradually reduced at each down sampling step, and feature maps of each size were concatenated in the corresponding up-sample layer to extract the hierarchy of spatial information. Meanwhile, time-series feature maps of images with moderate to high spatial resolution were extracted by two consecutive 3×3 convolutional layers and a 1×1 convolutional layer. Then, the time-series and spatial feature maps were integrated via element-wise addition before they were sent to the decoder for pixel-wise classification.Evaluation results from randomly selected sample points showed that CEUNet achieved good performance with an overall accuracy of 92.92% over the whole of Qianjiang City, Hubei Province. The CEUNet-extracted cropland at the meter-level resolution was used to perform a wall-to-wall pixel comparison with the cropland extracted via semantic segmentation based on UNet by using multi-source remote sensing images with different resolutions (UNet_m). Semantic segmentation based on UNet using single-phase high-resolution images (UNet_s), object-based random forest classification (OBIA), and pixel-based random forest classification (RF) were employed to extract the results of cultivated land for comparison. The accuracy of cropland extraction by CEUNet was higher than that by others (the average F1 score was improved by about 0.04, 0.11, 0.21, and 0.21), indicating the effectiveness of the proposed approach for diverse agriculture landscapes. The F1 score of CEUNet was improved by about 0.09, 0.26, 0.27, and 0.27 over regions with high fragmentation and complex landscapes.