下载中心
优秀审稿专家
优秀论文
相关链接
首页 > , Vol. , Issue () : -
摘要
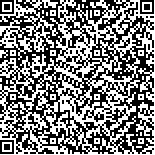
遥感作为大范围地表覆盖提取和时空动态监测的有力工具,在小麦种植面积反演领域具有显著优势。然而,混合像元现象限制了小麦种植面积估计的精度,而端元的光谱变异性现象也使得传统的混合像元分解方法表现不佳。针对小麦种植面积估计过程中存在的混合像元和端元光谱变异性等问题,本文提出基于自监督学习的光谱解混算法 (Self-supervised Learning-based Spectral Unmixing Algorithm, SLSU)。首先,使用变分自编码器实现无监督的端元光谱变异性解释和端元库生成;其后,使用交替最小二乘法和全约束最小二乘模型估计各类端元对应的丰度;最后,利用概率松弛标记法对解混结果进行空间邻域校正,以进一步提高光谱解混和小麦面积估计的精度。以河南省新乡市3个典型的小麦种植区为实验区,利用Sentinel 2影像获取小麦种植面积并利用实地测量的小麦分布现状数据计算提取精度。结果表明:基于SLSU算法获取的小麦种植面积的相对提取误差中位数小于1.3个像素,显著优于全约束最小二乘、扩展线性混合模型等传统混合像元分解算法以及支持向量机、随机森林等监督学习分类方法所提结果。所提SLSU算法可以提高小麦提取的精度和稳定性,为农作物分布提取和种植面积估算提供了有效的方法。
Wheat is an important food crop in China, and its distribution acquisition and planting area estimation are of great significance to ensure national food security and development planning. As a powerful tool for large-scale land cover extraction and temporal and spatial dynamic monitoring, remote sensing has significant advantages in the field of wheat planting area estimation. However, wheat is often mixed with other ground objects and forms mixed-pixels limited by the spatial resolution of remote sensing images and the influence of various factors in the transmission of electromagnetic radiation. The spectral mixing limits the accuracy of wheat planting area estimation. Meanwhile, endmember spectral variability also makes tradi-tional spectral unmixing methods perform poorly. Thus, this paper proposes a Self-supervised Learning-based Spectral Unmixing Algorithm (SLSU) to alleviate the influence of spectral mixing and endmem-ber spectral variability wheat planting area estimation. Firstly, the variational autoencoder is used to achieve unsupervised interpretation of the endmember spectral variability and endmember library generation. Then, abundances corresponding to various endmembers are estimated by using the alternating least-squares strategy and fully constrained least squares. Finally, the unmixed results are corrected based on the spatial neighborhood by using the probabilistic relaxation labeling algorithm to further improve the accuracy of spectral unmixing and wheat extraction. Three typical wheat planting areas in Xinxiang, Henan Province, were selected as experimental areas, and the wheat planting area was obtained by Sentinel 2 images. The extraction accuracy was evaluated by wheat distribution data measured in the field, and the re-sults show that the median value and the R2 score of the wheat extraction’s relative extraction error are close to 1.3 pixels and 1.00, respectively, which are significantly better than the results extracted by traditional spectral unmixing algorithms (including fully constrained least squares, extended linear mixed model and so on) and traditional supervised learning classification methods (such as support vector machines and random forests). The proposed SLSU algorithm can improve the accuracy and stability of wheat plant-ing area estimation and provide an effective method for crop distribution extraction and planting area esti-mation.