下载中心
优秀审稿专家
优秀论文
相关链接
摘要
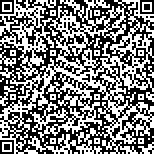
Landsat系列、ASTER等中等空间分辨率遥感数据(中分数据)覆盖了碳酸盐矿物、粘土矿物、铁氧化物矿物等矿物的诊断光谱区间,广泛应用于矿物、岩石信息提取,但受限于空间分辨率,混合像元现象明显,严重制约了其岩性分类精度。WorldView-2、QuickBird等高空间分辨率遥感数据(高分数据)提供了岩石地层表面丰富的空间结构信息,同时空间分辨率的提高也是缓解混合像元效应的最有效途径,但高分数据覆盖的光谱区间往往较窄,难以满足大多数特征吸收谱段位于短波红外、热红外区间的矿物、岩石信息提取。在岩性自动分类方法上,前人研究中仍以采用基于像元的分类方法为主,分类结果的“椒盐现象(Salt-and-pepper,出现在分类结果图中大量孤立的错分点或小图斑)”严重。为结合中分数据的光谱信息优势和高分数据的空间结构信息优势,同时减少基于像元的岩性分类方法中的“椒盐现象”,提高岩性自动分类精度,本文以Landsat 8 OLI数据和WorldView-2数据为例,提出了一种协同中、高分遥感数据进行面向对象的岩性模糊分类的方法。首先通过“结构协同”和“光谱协同”方案对WorldView-2数据和OLI数据进行信息协同,利用主成分变换对协同后数据的纹理信息和光谱信息进行压缩和增强,然后将增强后的纹理信息和光谱信息进行波段绑定,并进行多尺度分割。根据岩性单元间的光谱特征和纹理特征的差异,构建各岩性单元的模糊逻辑隶属度函数,实现对研究区岩性的模糊分类。实验结果表明,该方法成功划分了岩性单元的分布,总体岩性分类精度为89.35%。
关键词:
遥感 WorldView-2 Landsat 8 OLI 特征协同 岩性 模糊分类Medium spatial resolution data, such as TM (Theme Mapper), ETM+ (Enhanced Theme Mapper Plus), OLI (Operational Land Imager) and ASTER (Advanced Spaceborne Thermal Emission and Reflection Radiometer), has been widely applied in lithological mapping and minerals mapping, because of covering the diagnostic spectral regions of carbonate rocks, clay minerals, iron oxide minerals, etc. However, due to the relatively coarse spatial resolution, the phenomenon of mixed pixels is obvious, which severely restricts the accuracy of lithological mapping of medium spatial resolution data. High spatial resolution data, such as WorldView and QuickBird, provides rich spatial structure information of rock surfaces. Meanwhile, the improvement of spatial resolution is also the most effective way to alleviate the phenomenon of mixed pixels, but the spectral range of high spatial resolution data is often narrow and difficult to extract of minerals and rocks with characteristic reflectance absorption in short-wave infrared and thermal infrared regions. And, for lithological classification method, pixel-based classification methods are still mainly used in previous studies, exhibiting the undesired “salt-and-pepper” phenomenon.The objectives of this study are to (1) combine and enhance the spectral information and spatial structure information of high spatial resolution data (WorldView-2) and medium spatial resolution data (OLI) and (2) evaluate fuzzy classification method for lithological mapping. Firstly, WorldView-2 data and OLI data were structural and spectral combined. Then, texture information and spectral information of the combined data were compressed by PCA, compressed texture layer and compressed spectral layers are selected and stacked. The feature combined data was multi-scale segmented. Finally, the fuzzy logic membership functions of the rock types were built, based on the texture and spectral difference of rock types. And the lithological fuzzy classification of study area was carried out.Results showed that the proposed method classifies the rock types of study area successfully, and received a high total accuracy of 89.35%.