下载中心
优秀审稿专家
优秀论文
相关链接
摘要
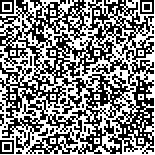
红树林是世界上生产力最高、价值最高的湿地生态系统之一。冠层叶绿素含量CCC(Canopy Chlorophyll Content)作为红树林重要的生物物理参量,是估算其生产力和评价其健康状况的重要指标。本文利用珠海一号高光谱卫星(OHS)影像与Sentinel-2A多光谱数据计算传统植被指数与组合植被指数并构建了高维数据集,综合利用正态分布检验、最大相关系数法与变量重要性评价进行数据降维和变量优选;分别基于单一线性回归算法、机器学习回归算法和堆栈集成学习回归算法构建了红树林CCC遥感反演模型,探明北部湾红树林CCC的最佳遥感反演模型,验证OHS高光谱影像与Sentinel-2A数据反演红树林CCC的精度差异,评估SNAP-SL2P算法反演红树林CCC的适用性。研究结果表明:(1)通过数据降维和变量选择处理,从高维度OHS数据集选取了8个特征变量,其中RSI(12,17)、DSI(12,18)和NDSI(6,12)组合植被指数对红树林CCC反演精度的贡献率较高;(2)联合OHS数据和最优堆栈GBRT集成学习回归模型(Score=0.999,RMSE=0.963 μg/cm2)的训练精度优于最优RF机器学习回归模型(RMSE降低了7.531 μg/cm2),明显优于最优Lasso线性回归模型(RMSE降低了19.383 μg/cm2);(3)在最优堆栈集成学习回归模型下,OHS数据反演红树林CCC的精度(R2=0.761,RMSE=16.738 μg/cm2)高于Sentinel-2A影像(R2=0.615,RMSE=20.701 μg/cm2);(4)联合OHS和Sentinel-2A数据的最优堆栈集成学习回归模型反演红树林CCC的精度都明显优于SNAP-SL2P算法(R2=0.356,RMSE=49.419 μg/cm2)。研究结果论证了正态分布检验、最大相关系数法和基于XGBoost的特征选择方法有效降低了高维数据集的维度,并得到了最优特征变量;OHS数据的最优堆栈GBRT集成学习回归模型训练精度最高,是估算红树林CCC的最优反演模型;OHS和Sentinel-2A数据都能有效反演红树林CCC(R2均大于0.61),而OHS数据的估算精度更高(R2大于0.75);SNAP-SL2P算法不能有效反演红树林CCC(R2小于0.4),且对红树林CCC数值存在系统性低估。
Mangroves are one of the most productive and valuable wetland ecosystems in the world. Canopy Chlorophyll Content (CCC), as an important biophysical parameter, is an important indicator to evaluate mangroves’ productivity and health status.This study calculated traditional vegetation index, combined vegetation index using Zhuhai-1 Hyperspectral Satellite (OHS) and Sentinel-2A multispectral images, and produced high-dimensional datasets. Dimension reduction and variable selection were carried out by combining normal distribution test, correlation analysis, and importance evaluation of feature variables. The optimal inversion model of mangrove CCC in the Beibu Gulf was built using single linear regression, machine learning regression, and stacking ensemble learning regression algorithm. This study demonstrated the inversing accuracy difference between OHS image and Sentinel-2A data, and evaluated the applicability of SNAP-SL2P algorithm in mangrove CCC inversion.Results showed that (1) eight optimal feature variables are selected from the high-dimensional datasets of OHS image by statistical test, maximum correlation coefficient, and variable importance evaluation. Combining vegetation indexes (RSI(12,17), DSI(12,18), and NDSI(6,12)) highly contributes to the inversion of mangrove CCC. (2) The training accuracy of the GBRT-based optimal stacking ensemble learning regression model using OHS data (Score=0.999, RMSE=0.963 μg/cm2) was higher than that of the optimal RF regression model (RMSE reduced by 7.531 μg/cm2), which is better than the optimal Lasso linear regression model (RMSE reduced by 19.383 μg/cm2). (3) The inversion accuracy of the optimal stacking ensemble learning regression model using OHS data (R2=0.761, RMSE=16.738 μg/cm2) is higher than that from Sentinel-2A image (R2=0.615, RMSE=20.701 μg/cm2). (4) The optimal stacking ensemble learning regression model using OHS and Sentinel-2A data in estimating mangrove CCC outperforms the SNAP-SL2P algorithm (R2=0.356, RMSE=49.419 μg/cm2).The conclusion demonstrated that normal distribution test, maximum correlation coefficient method, and XGBoost-based feature selection method can effectively reduce the redundancy of high-dimensional datasets, and obtain the optimal feature variables. The optimal stack GBRT ensemble learning regression model with the OHS data has the highest training accuracy, which is the optimal inversion model for estimating CCC of the mangrove. The R2 of OHS and Sentinel-2A data is over 0.61, which indicated that OHS and Sentinel-2A data can effectively estimate mangrove CCC. SNAP-SL2P algorithm cannot effectively inverse mangrove CCC (R2 is less than 0.4) and systematically underestimates CCC value.