下载中心
优秀审稿专家
优秀论文
相关链接
摘要
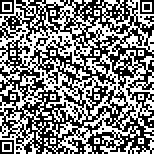
科学准确地监测红树林是保护海陆过渡性生态系统的基础和前提,但红树林分布于潮间带,难以进行大规模人工监测。遥感技术能够对红树林进行长时间、大面积监测,但已有研究尚存不足。一方面,红树林分布于热带、亚热带区域,受到天气条件限制难以获得长时间覆盖的有效光学遥感数据;另一方面,红树林极易与其他陆生植被混淆,仅利用多波段数据的光谱信息难以精确识别。本文以恒河三角洲孙德尔本斯地区为例,基于谷歌地球引擎GEE(Google Earth Engine)获取2016年全年的Landsat 8 OLI和Sentinel-2 MSI数据,利用物候信息进行红树林提取研究。首先,基于最小二乘回归构建两个传感器在相同指数之间的关系,重建时间序列数据,之后根据可分性判据选取增强型植被指数EVI(Enhanced Vegetation Index)和陆地表面水分指数LSWI(Land Surface Water Index)。其次,对两个指数的时间序列数据进行Savitzky-Golay滤波处理,并分别提取生长期始期等13种物候信息。最后,将两个指数的物候信息进行特征级联,采用随机森林RF(Random Forest)方法进行分类,提取研究区红树林范围。实验结果表明:Landsat 8 OLI和Sentinel-2 MSI数据融合可有效提升时间序列质量,与基于单一传感器数据的分类结果相比,总体精度提高1.58%;物候信息可以显著增强红树林与其他植被的可分性,与直接使用时间序列数据的分类结果相比,总体精度提高1.92%;同时考虑EVI和LSWI指数可极大地提升分类效果,与采用单一指数相比,总体精度分别提高14.11%和9.69%。因此,本文通过数据融合、物候信息提取和指数特征级联可以更好地提取红树林,总体精度达到91.02%,Kappa系数为0.892。研究验证了物候信息在红树林遥感监测中的应用潜力,提出的方法对科学准确地监测全球或区域红树林具有一定参考价值。
Scientific and accurate monitoring of mangroves is the basis and premise for the protection of marine coastal and transitional ecosystem. However, mangroves are mainly distributed in the intertidal zone, result in large-scale manual monitoring a tough task. Although remote sensing technology can map mangrove within for long time and large area, the existing studies have some shortcomings. On the one hand, mangroves are often distributed in tropical and subtropical regions, where long-term coverage of effective optical remote sensing data is difficult to obtain due to weather conditions. On the other hand, mangroves are easily confused with other terrestrial vegetation by only using spectral information.In this paper, we choose the Sundarbans located in the Ganges River Delta as the study area. Landsat 8 OLI and Sentinel-2 MSI data in 2016 are obtained based on GEE (Google Earth Engine) to conduct mangrove extraction in this research. Firstly, the relation between the two sensors for the same index is constructed based on a least square regression model, which is used to reconstruct the time series data. In this phase, EVI (Enhanced Vegetation Index) and LSWI (Land Surface Water Index) are selected according to the separability criterion. Secondly, Savitzky-Golay filtering is applied to the time series data of the two indices, and 13 phenology metrics are extracted. Finally, these metrics of the two indices are cascaded, and Random Forest (RF) is used to extract the area of mangrove.Fusing the Landsat 8 OLI and Sentinel-2 MSI can effectively improve the quality of time series data. Compared with the classification results based on single sensor data, the overall accuracy is improved by 1.58%. Phenology information can significantly enhance the separability between mangrove and other vegetation, with a 1.92% improvement of overall accuracy compared with the classification results using time series data directly. Considering both EVI and LSWI indices can greatly improve, the classification effect, with 14.11% and 9.69% improvements compared with using a single index. Therefore, the method in this paper can effectively extract mangroves, with the overall accuracy and Kappa coefficient reaching to 91.02% and 0.892 respectively.This research takes full account of the deficiency of optical remote sensing monitoring, biological characteristics and geographical characteristics of mangroves, which can extract the area of mangroves more objectively and accurately. Compared with other similar studies, the differences and characteristics of this study are: (1) Jointly using EVI and LSWI time series to describe the phenological information of mangroves can effectively differentiate mangroves and evergreen forests; (2) We introduce phenology information into mangrove classification using remote sensing for the first time, and verify the feasibility of using phenology information to monitor the range of mangrove. The method proposed in this paper may be benefit for scientific and accurate monitoring of global or regional mangroves.