下载中心
优秀审稿专家
优秀论文
相关链接
摘要
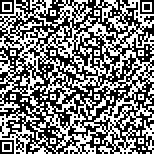
受仪器和观测条件限制,高光谱数据易受噪声污染,给数据解译带来挑战。针对传统稀疏解混模型抗噪性能差的问题,本文提出一种截断加权核范数稀疏解混方法,利用高光谱图像像元之间的相关性减轻噪声对丰度估计的干扰。该方法借助低秩表示在挖掘数据内在低维结构方面的优势,在稀疏解混中加入基于截断加权核范数的低秩约束,并结合加权稀疏技术,在稀疏正则项中引入空间邻域权重。截断加权核范数对丰度矩阵的奇异值向量分段处理,可以更好地实现丰度矩阵的低秩逼近,使丰度图像保持空间一致性并保留更多细节信息,空间加权策略则增强了丰度图像的空间连续性。模拟高光谱数据、Cuprite矿区真实数据和红树林高光谱数据实验表明,与其他先进的稀疏解混方法相比,所提方法具有更好的抗噪性,能够提高解混精度。
Spectral unmixing is an important technology for quantitative analysis of hyperspectral images, which estimates the pure source signal (endmember) and the corresponding fractional proportion (abundance). Sparse unmixing is one of the research highlights in the field of spectral unmixing. Sparse unmixing finds a set of endmembers that can optimally model mixed pixels from a known spectral library and takes the fractional abundance as the weight, thereby circumventing the process of endmember extraction. However, hyperspectral data are often contaminated by noise due to the limitations of instruments and observation conditions. This state is disadvantageous to data interpretation. Sparse unmixing is peculiarly prone to be disturbed by noise and thus affect the accuracy of abundance estimation or even erroneously identify endmembers from spectral libraries.To overcome this drawback, this study proposes a hyperspectral sparse unmixing method with truncated weighted nuclear norm, which exploits the correlation of pixels to reduce the interference of noise on abundance estimation. The proposed method adds the low-rank constraint based on truncated weighted nuclear norms to the sparse unmixing model given that low-rank representation is available to mine the inherent low-dimensional structure of data. It is different from other nuclear norm minimization, singular values are divided into two groups and treated with the truncated nuclear norm and weighted kernel norm. It provides a better low-rank approximation of the abundance matrix, which maintains the spatial consistency of image and protects the detailed information. Inspired by the weighted sparse strategy, the spatial neighborhood weight is introduced into the sparse regularization term, which enhances the spatial continuity of image. The underlying optimization problem is solved by the alternating direction method of multipliers efficiently.Experiments are conducted on simulated data, real Cuprite, and mangrove hyperspectral data to verify the unmixing performance of the algorithm. In particular, there is no available spectral library for mangrove hyperspectral data, which is essential for the sparse unmixing algorithm, so a spectral library is built derived from the original data. The various vegetation curves in the library are relatively close, which brings challenges to the unmixing task. Even so, the proposed method identified all mangrove species, and achieved approximately consistent results with the reference classification map. Compared with other advanced sparse unmixing methods, the proposed method is superior in restraining the influence of noise and can obtain high unmixing accuracy even in the case of high noise.In future work, we will further explore the spatial information of hyperspectral images with tensor-based low-rank representation to improve the robustness of the sparse unmixing algorithm. In addition, we will collect hyperspectral data with more mangrove species and expand the corresponding spectral library, further develop mangrove species classification techniques based on spectral unmixing to better serve the investigation of mangrove species composition.