下载中心
优秀审稿专家
优秀论文
相关链接
摘要
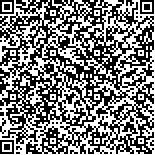
许多城市建立的相对稠密的网格化监测站点,为精细化监管城市空气质量奠定了基础。本文选用徐州市网格化监测数据、地球静止卫星Himawari-8/AHI及COMS/GOCI的表观反射率和气溶胶光学厚度数据、气象和其他辅助数据,开展了徐州地区0.005°空间分辨率网格的PM2.5浓度精细化制图研究。本文使用了极端梯度提升(XGBoost)、随机森林(RF)及时空加权回归(GTWR)等3种方法,并选用多种特征参数组合进行对比分析。综合分析模型精度和过拟合程度,结果表明XGBoost模型表现最好,其R2为0.90,RMSE为11.65 μg/m3。进一步将本文结果与国控站点、清华大学的TAP数据集和马里兰大学的CHAP数据集的对比分析,结果表明基于网格化站点的PM2.5制图结果能更好地反映城市内部不同区域的PM2.5浓度分布差异性,弥补因国控站点稀疏带来的缺陷,更好地服务于城市空气质量精准管控。
The dense gridded air quality monitoring sites established by local governments in China have laid the foundation for fine-tuned monitoring of urban air quality. However, whether it can help improve the capabilities of satellite-based surface PM2.5 concentration estimation and mapping remain to be tested. To this end, we conducted a case study with Xuzhou as an example.In order to describe the spatial distribution of PM2.5 in detail at urban scale , this study used the PM2.5 concentration data from 172 grid monitoring stations in Xuzhou, the apparent reflectance and aerosol optical thickness data from the Himawari-8/AHI and COMS/GOCI, meteorological data from ERA5 and other auxiliary data to carry out fine mapping study of PM2.5 concentration on the 0.005° spatial resolution grid. The machine learning and geostatistical algorithms of eXtreme Gradient Boosting (XGBoost), Random Forest (RF) and Geographically and Temporally Weight Regression (GTWR) are applied, and a variety of characteristic parameter combinations (listed in the body of the paper) were selected for comparative analysis.Compared with other two prediction models, the XGBoost model performed the best, in terms of model accuracy and degree of overfitting, with high correlation coefficient (0.90) and low root mean squard error (11.65 μg/m3). Meanwhile, the best parameter combination includes satellite data from Himawari-8 as well as GOCI and meteorological data from ERA5. These retrieved results were then compared with the measurements obtained from the national grid monitoring stations. For peer comparison, in addition, the TAP (Tracking Air Pollution in China) dataset of Tsinghua University and the CHAP (China High AirPollutants) dataset of the University of Maryland are also involved in this comparison, in the aspect of daily mean value and capacity of fine mapping of PM2.5 in Xuzhou. Besides, these two datasets use the data from national grid monitoring stations as the validation data..The following conclusions can be drawn:(1) Due to its higher temporal resolution, geostationary satellite measurements can better fit hourly urban grid monitoring station data in model prediction, and is more suitable for urban PM2.5 fine mapping than polar orbit satellite;(2) The estimation results based on urban grid monitoring stations and satellite measurements can make up for the lack of sparse national control stations to a certain extent, better reflect the difference of PM2.5 concentration distribution between different regions within a city, and better serve the accurate control of air pollution.