下载中心
优秀审稿专家
优秀论文
相关链接
摘要
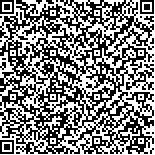
码头自动识别能够为港口的建设与开发、海岸带地理信息的获取及海上军事实力的评估提供重要依据。然而由于码头普遍尺寸小、数量多、分布散乱,且受周围船舶、建筑等环境干扰严重,传统算法难以满足对高速发展的码头进行准确监测的需求,如何对码头目标进行准确识别成为亟需解决的问题。本文基于公开遥感数据集及Google Earth高分遥感影像构建了3种码头类型的数据集,并针对码头的尺寸特征和空间分布特征对Faster R-CNN算法进行了如下改进:(1)采用K-Means算法对候选框进行预设,使其大小更适应码头尺寸;(2)采用Soft-NMS算法代替NMS算法,以降低分布密集地区码头的误删率和漏检率。实验结果表明,本文改进的Faster R-CNN算法FKSN(Faster R-CNN+K-Means+Soft-NMS)识别精度达到92.6%,相较Faster R-CNN算法精度提高了8.3%。将码头目标识别结果和传统分类方法ISODATA、SSD及Faster R-CNN、Faster R-CNN+K-Means等目标提取模型的识别结果相对比,本文方法在虚警率和漏检率的评价指标表现最好,分别为3.2%和7.6%,说明本文方法对于各类码头目标识别具有更好的效果。基于改进Faster R-CNN算法的码头自动识别研究可以为码头的合理建设、规划及治理提供技术支持,为港口高效利用和军事实力分析提供有效途径。
Automatic identification of docks can provide an important basis for the construction and development of ports, acquisition of coastal geographic information, and evaluation of maritime military strength. However, docks are characterized by small sizes, large quantities, and scattered distribution. Docks are also negatively affected by serious information interference of the surrounding environment, including ships and buildings. Traditional algorithms cannot easily meet the needs of accurate monitoring of rapidly developing docks. Accurate identification of dock targets has become an urgent problem to be solved. Based on the open remote sensing data sets and Google Earth high-resolution remote sensing images, the data sets of three types of docks are constructed, and the following improvements are made to the Faster R-CNN algorithm according to the size and spatial distribution characteristics of docks. (1) The K-means algorithm is used to preset the anchors, making the anchor sizes more suitable for the actual dock sizes. (2) Soft-NMS is used instead of NMS to reduce the rates of mistaken deletion and missed detection of dock borders in densely distributed areas. The experimental results show that the accuracy of the improved FKSN algorithm reached 92.6%, which is 6% higher than that of the Faster R-CNN algorithm. The final result of dock target recognition is compared with the ones of the traditional classification methods such as ISODATA, SSD, Faster R-CNN, and Faster R-CNN+K-Means. Among these approaches, the method suggested in this paper performs best in the evaluation indices of false alarm rate and omission rate, which are 3.2% and 7.6%, respectively. Thus, the proposed method has a better effect on the identification of various dock targets. The automatic dock identification algorithm based on the improved Faster R-CNN can provide technical support for reasonable construction, planning, and management of docks and provide effective approaches for efficient utilization and military strength analysis of docks.