下载中心
优秀审稿专家
优秀论文
相关链接
摘要
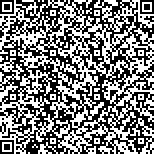
地表温度对于全球气候变化等研究具有重要意义。被动微波遥感传感器AMSR-E(Advanced Microwave Scanning Radiometer for EOS)可以获得全天候地表温度,可作为多云条件下热红外地表温度数据的补充;但轨道扫描间隙限制了该数据在全球或区域尺度上的实际应用。鉴于地表温度的高时空异质性和AMSR-E LST轨道间隙数据的特点,本文提出了一种多时相特征连接卷积神经网络地表温度双向重建模型(MTFC-CNN),利用深度学习在处理复杂非线性问题上的优势,重建轨道间隙区域的地表温度值。将2010年中国大陆四季的AMSR-E LST数据(数据未含港澳台区域),分为白天和夜晚,形成共8个数据子集进行实验。在模拟实验中,重建结果与原始反演地表温度值平均均方根误差在1.0 K左右,决定系数R2在0.88以上,优于传统的样条空间插值和时间线性回归方法;真实实验结果具有较好的目视效果,且与对应MODIS LST产品对比发现,重建区LST值和未重建区LST值与MODIS LST产品间具有相近的平均均方根误差和决定系数。因此,本文提出的MTFC-CNN方法能有效重建AMSR-E LST轨道间隙数据,且优于传统方法。
Land Surface Temperature (LST) is a key parameter in the physical process of surface radiant energy balance and the water cycle. Obtaining LST data accurately and promptly, and mastering its temporal and spatial changes, are of considerable importance to climate change research. Thermal infrared (TIR) measurements are limited in practical applications due to cloud cover and other effects. Passive microwave (PMW) remote sensing measurements can penetrate clouds and are less affected by atmospheric interference, which has the advantage of obtaining all-weather surface radiation information. The microwave remote sensing data Advanced Microwave Scanning Radiometer for EOS (AMSR-E) can obtain all-weather LST, which can be used as a supplement to the missing LST information in thermal infrared (TIR) products under cloudy conditions. However, the AMSR-E data has the problem of lack of information due to the satellite orbit scanning gap of its own sensor, causing the obtained AMSR-E LST data to be greatly restricted in practical applications. Therefore, proposing an effective method for solving the problem is necessary.Based on the superiority of deep learning in solving non-linear problems and the high dynamic variability of LST, this paper proposes a Multi-Temporal Feature-Connected Convolutional Neural Network (MTFC-CNN) that uses specific input combinations of multi-temporal information and spatial fusion units. The network structure is based on the characteristics of the temporal and spatial distribution of missing track gaps in AMSR-E LST data, and the reconstruction of missing LST values is carried out from the timing information.In the simulation experiment, the 2010 annual data was divided into fight data subsets in four seasons and into day and night. The average root mean square error of the reconstructed LST value is approximately 1.0 K and the coefficient of determination R2 is above 0.88. Compared with the other two methods, namely, spline interpolation (Spline) and time multiple linear regression (Regress), the reconstruction effect of the MTFC-CNN method performs better regardless of seasons or day and night, proving that MTFC-CNN is better than the other two methods at mining the characteristics of temporal and spatial changes in LST. In real experiments, through comparison with MODIS LST products, the LST value reconstructed at the missing area is consistent with it at other areas in temporal and spatial distribution. The reconstruction results show that the LST in the mainland China region shows a gradually increasing trend from January to July and a gradually decreasing trend from August to December. This finding is consistent with the temperature changes in the four seasons. The change in LST during the day is more significant than that at night. In summer, the temperature in Northwest China is significantly higher than that in other regions. In winter, the temperature in Northeast China is generally lower than that in other regions. At night, the difference between summer and winter is more obvious. The difference in LST changes at night in autumn is relatively close.The experimental results show that the MTFC-CNN method proposed in this paper mines the spatio-temporal variation information of LSTs more effectively than two traditional methods and achieves better results in reconstructing the orbital gap missing in AMSR-E LST data. The method provides the possibility for the reconstruction of missing information from TIR LST data under the cloud.